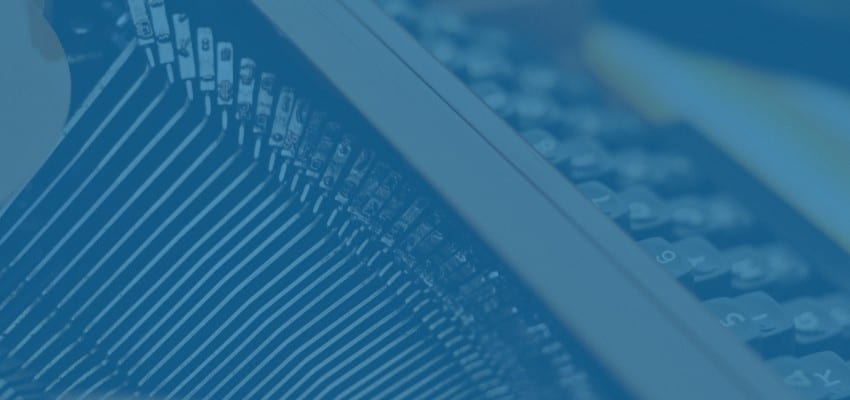
[Webcast Transcript] The Only Thing Constant is Change: An eDiscovery Technology and Tools Update
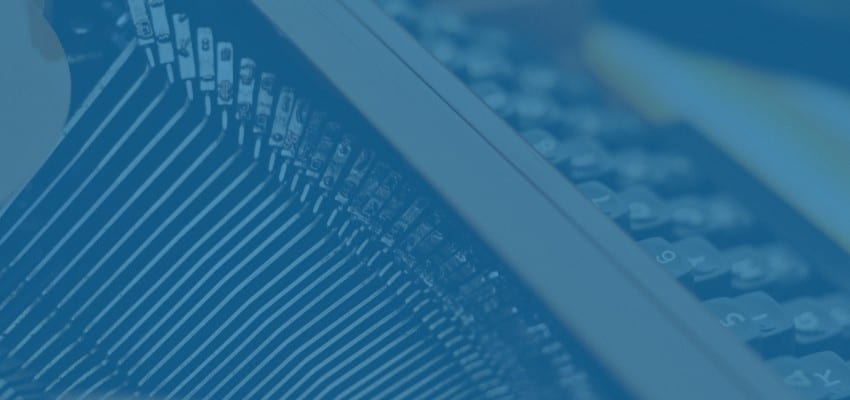
Editor’s Note: On May 20, 2020, HaystackID shared an industry update of current technologies for data analytics, artificial intelligence, process automation, and data enhancement. While the full recorded presentation is available for on-demand viewing via the HaystackID website, provided below is a transcript of the presentation as well as a PDF version of the accompanying slides for your review and use.
The Only Thing Constant is Change: An eDiscovery Technology and Tools Update
Today, standardized collection, processing, and review processes and protocols are becoming commonplace. However, the technologies and tools that provide efficiencies and enhancements to these eDiscovery processes and protocols are continuing to evolve.
In this presentation, expert eDiscovery technologists and authorities will provide an overview of current technologies for data analytics, artificial intelligence, process automation, and data enhancement. They will also offer practical considerations for implementing current technologies and evaluating emerging technologies.
Webcast Highlights
+ A View from the Bench: How the Courts Are Considering New Technologies
+ Considering Forensic Analysis: New Technologies and Approaches
+ Beyond Tradition: An Update on Audio, Video, and Foreign Language Analysis
+ Emerging Data Types: Analyzing the New With the Latest Tools
+ Faster Review and Faster Decisions: Enhancing Document Review
Presenting Experts
Ashish Prasad
As VP and GC for HaystackID, Ashish is regarded as among the leading experts on discovery in the US. He has served as Litigation Partner, Founder and Chair of the Mayer Brown LLP Electronic Discovery and Records Management Practice, Founder and CEO of Discovery Services LLC, and VP and GC of eTERA Consulting.
Todd Haley
As Executive Vice President and General Manager for eDiscovery Operations for HaystackID, Todd provides consulting and operational guidance to help legal departments and law firms address matters related to the planning, management, and execution of eDiscovery.
Vazantha Meyers, Esq.
As Vice President of Managed Review for HaystackID, Vazantha has extensive experience in advising and helping customers achieve their legal document review objectives.
Presentation Transcript
Introduction
Hello, and I hope you’re having a great week. And we wish you health and safety for both you and your loved ones during this challenging time. My name is Rob Robinson. And on behalf of the entire team at HaystackID, I’d like to thank you for attending today’s webcast titled, The Only Thing Constant is Change, an eDiscovery Technology and Tools Update.
Today’s webcast is part of HaystackID’s monthly series of educational presentations conducted on the BrightTALK Network and designed to ensure listeners are proactively prepared to achieve their computer forensics, eDiscovery, and legal review objectives during investigations and litigation.
Our expert presenters for today’s webcast include three of the industry’s foremost subject matter experts and the authorities on eDiscovery. Those experts being Ashish Prasad, Todd Haley, and Vazantha Meyers.
Our first presenter is Ashish Prasad, HaystackID’s vice president, and the general counsel for HaystackID. He’s widely regarded as one of the leading experts on eDiscovery in the United States and has served in leading industry roles to include litigation partner, founder and chair of the Mayer Brown Electronic Discovery and Records Management Practice, vice president and general counsel of eTERA Consulting, founder and CEO of Discovery Services, and also served as an adjunct professor of law at Northwestern University Law School where he teaches advanced topics in litigation and civil discovery. And in addition to having extensive experience in managing all aspects of discovery and litigation and investigations, Ashish has defended compliance procedures before numerous courts and regulators.
Our second expert presenter is Todd Haley, an executive vice president and GM for eDiscovery operations at HaystackID. Todd provides consulting and operational Guidance to help legal departments and law firms address matters related to the planning, management, and execution of eDiscovery. An information technology expert with industry-acknowledged expertise in managed services, Todd has served in IT and leadership positions with multiple law firms and technology integrators.
Our final presenting expert is Vazantha Meyers. Vazantha serves as vice president of Discovery Services for HaystackID. She has extensive experience in advising and helping customers achieve their legal document review objectives. And she’s recognized as an expert on all aspects of traditional and technology-assisted review. She’s a graduate of Purdue University and obtained her JD from Valparaiso University School of Law.
Today’s presentation will be recorded for future viewing. And a copy of the presentation materials are available for all attendees. And you can access these materials directly beneath the presentation in viewing the window on your screen by selecting the Attachments tab on the far left of the toolbar beneath the viewing window.
And as with all of our webcasts, this webcast will be made available for on-demand viewing directly following the live presentation from both the HaystackID website and from the BrightTALK network. And at this time, I’d like to turn the presentation over to our expert presenters led by Ashish Prasad. Ashish.
Ashish Prasad
Thank you, Rob, and good afternoon, everyone. It is a pleasure to join you today. I’ll be talking about a view from the bench with respect to new eDiscovery technology. Let me begin by giving a special thanks to the large number of federal judges, as well as state judges and federal and state regulators who’ve made the time to join our seminar today.
It really means a lot to those of us in the private sector that those of you on the bench and in the government in regulatory roles are taking the time and making the effort to learn about eDiscovery technology and the challenges that technology poses for people like us in the private sector, so thank you.
Stepping back at the highest level, eDiscovery is generally understood as an activity that occurs at the intersection of law, technology, and business process. Our goal in eDiscovery is always to achieve defensibility of process with courts and regulators and efficiency of process for the protection of our corporate clients and law firms.
To achieve defensibility of process and efficiency, I think we have to recognize where in the law in the technology and in the business process the risks lie so that we can mitigate those risks. Most of the law of eDiscovery, as most people of this call know, has become largely settled over the past five years. There are advances in the law to be sure. But in terms of the legal rules that we need to operate under, most of that has been largely settled over the past five years.
The same is probably true on the topic of business process. While it is true that not as many corporate legal departments and law firms are following the most efficient business processes in eDiscovery as we would like, it is also true that we know, generally, what those efficient business processes are and that action is really in implementing what we know to be good processes and particular matters. That’s a very big challenge, which I think is very well-known and familiar to the large number of corporate legal department attendees on our seminar today.
Today, we’re drilling into what I view as the biggest risk-generating of the three-legged stool of eDiscovery. And that is technology. Technology in eDiscovery is changing very rapidly. And it has been that way for the past decade. And it is likely to be that way for the coming decade. We simply cannot in eDiscovery assume, as everyone in the world likes to assume, that the future will be like the past.
So in this seminar today, we’re going to be talking about technology changes in eDiscovery and what those changes mean for practitioners in the area– be they in government agencies or corporate legal departments or law firms or even service providers, like HaystackID ID. The one point I’d like to make before we get into the specific technologies that we’ll be discussing is that we don’t have that much direct Guidance from the judges, nor from the regulators on some of the technology changes that we are seeing in eDiscovery.
And we don’t have that Guidance for a very good reason. There’s no way that the courts and regulators can keep up with changes in technology. So what we’re left in the private sector to do is to manage the technology changes within the framework of defensibility of process and efficiency of process for the benefit of our client.
So what I think we should do is turn to talk about the specific technologies. But then as each of you are hearing about those technologies, I would ask you to think about them in the context of your own activities in eDiscovery. And in terms of maximizing defensibility of process and efficiency of processes.
So if anybody has any questions about that, please submit them on the chat line. And we’ll be sure to answer them. With that, let me turn it over to Todd Haley to talk about the changes in forensic technology. Todd?
Todd Haley
Thank you, Ashish. So today, yes, we’re going to start with forensic analysis. And we’re going to look at a couple of different tools in forensics that really aren’t about the mainstream ones. We obviously have Cellebrite. We have Guidance and some other things that handle the collection and analysis across forensics.
But what we’re really looking at today is also more nimble, more agile technologies that are allowing us to move as quickly as the technology does. In talking to our forensic experts, what we have determined is that many of the tools that have been identified are related to new media and new technologies.
The first one now called Magnet’s Axiom, which was formerly the Internet Evidence Finder, this allows for components of the new internet artifacts to become easily and quickly. And because of the agileness of the product, they’re able to adapt and add new things as new things are needed much more quickly than in the past.
Additionally, social discovery or social media is constantly changing to the point where the connectors for those products are changing approximately every quarter if not quicker. So tools such as X1 Social Discovery are able to keep up with that by making different changes to their products as those new medias change.
And then at the highest level, X1 and Apple products such as Blacklight and MacQuisition also are allowing for quick changes to those products that allow us to keep up with all of the technologies that are changing on a constant basis. If you think about it from this perspective, forensics has changed dramatically in the last five years in that the technologies that we’re dealing with, the emerging technologies, even the technology that has been around for a while, the technology life cycle has shortened to about a three-month lifecycle.
And so, these tools need to be able to keep up and adapt as those things occur. And so as you’re looking at emerging technologies and what’s happening, you want to make sure that you’re really working with forensic experts in order to work through some of these processes because what is good today may not be good tomorrow.
Even in the age of COVID-19, the requirements around certain tools have changed in that many of them used to be dongle-based, meaning that you had to have a particular hardware piece to attach to the computer. But with the requirement that forensic individuals have to do a lot more remote collections this year, a lot of them have even adapted to allow electronic licenses so that we can move more rapidly to remote locations worldwide.
The idea behind this is that emerging technologies create the need for speed, accuracy, and nimbleness. But in addition to forensics, we also have some of the other technologies that are becoming more mainstream in audio, video, and even foreign language. So with audio transcription, what we have determined is that the audio transcriptions are becoming more and more commonplace within discovery.
And one of the challenges was previously was that audio technology was really a single audio engine that you were trying to determine on how to read and transcribe that audio. With that single audio solution, you usually had anywhere from 30% to 50% accuracy. And many times, you didn’t have accurate transcripts across multiple languages.
Today, these emerging technologies have changed to allow for the ability to use multiple audio engines to provide more accurate data. The idea behind this is that those engines will have different components that will look at the same single audio source to determine what the language is, what the action is, and how that to look at that technology.
Additionally, we have these new technologies allow for the ability to train the engines using known audio verbiage to allow for all of the sounds to be calculated and counted. And then finally, it also allows for linguistic training that allows important words and phrases, especially around the industry, to be able to capture words that normally would not have been captured.
So if you think about it this way, you have five different audio sources. They are coming into a multi-engine solution. That multi-engine solution is looking at different things to analyze the sounds, the language, the component. And between those multiple languages, they get consolidated and then increase the accuracy of audio to 70% or above, allowing for a fairly good transcription of that audio.
The nice thing about this is that now with audio transcription becoming more and more accurate, not only can you take audio that you’ve identified in a process and listen to it, but it also can be transcribed in writing. And also, while you’re listening to it, it can highlight the words in the transcript to allow you to see the words while you’re listening to it.
Additionally, these technologies have also now allowed for you to search for those words within that written transcript and go directly to the location in the audio where that word or phrase is being used. The idea behind this is now that audio can be reviewed, tagged, and coded and produced similarly to written documents.
To add to that, we also have video transcriptions. If you think about it this way, today’s world, video is everywhere on iPhones where individuals are taking photos to security cameras within corporate networks to different body cams or CCTV. And video transcription is now becoming an emerging technology where video is becoming just as important as audio in looking at legal discovery.
Similar to audio transcription, this capability allows us, not only to proactively identify words with video for purposes of security and compliance and risk, but we can also use it in the legal industry to help legal teams to search, analyze, call, and explore across large amounts of video data in a platform that they’re already come to work in such as relativity or other products.
The nice thing about the video transcripts is it can work the exact same way that audio does in that you can get a written transcript of the video, do the search for the words, and identify a specific section of the video that you’re looking for and go right to it in that video.
But what happens when you have languages from all over the world? With a rapidly growing global community, these emerging technologies have to get better at understanding data across all languages, looking at different countries, different languages, and even different accents and dialects. The idea behind this is that language translation must emerge to the point where we can talk in a global community.
So how do these emerging languages technologies works? Well, the emerging technologies allow for neural networks, which have a much deeper learning translation and cover all the major language pairs of Europe, Asia, and the United States. The idea behind this is that by using language pairs, you’re able to identify languages, whether they have accents, whether they have specific regional dialects– things of that nature. And it allows for those languages to be interpreted and translated properly.
Additionally, these technologies allow for the training material to enhance those needs, especially when it comes to industry-specific words that may or may not translate normally or be found in regular translation technologies. By adding these industry-specific words to the training materials, you increase the ability of that language translator to translate those complicated scientific documents and things of that nature.
The nice thing also is today, that a lot of these language technologies allow for native-to-native translation. In the past, when the document was translated, it would be taken, for example, from a Word document and translated to a text document. Today, that native-to-native capability allows a Word document to remain a Word document and be translated into the new language.
This is also able to happen in Excel, PowerPoint, and even PDFs, which allows for it to stay more reasonably in its normal course of business while being translated into the language of choice. These technologies also work and integrate with major review platforms and become commonplace and allow those, both the original language and the translated language document to be linked together and reviewed together.
This allows for excellent workflows around machine language and around natural language for the purposes of translation. But they even go further than that. There are emerging technologies out there today that allow doctors to be coded in real-time in web-review platforms. When you code those documents, a few things can happen.
That coding can allow a document to be translated into multiple concepts. As you can see here, those concepts include the ability to have a human translator on the other side of the technology that’s doing full human translation. In addition, you can have that human translation plus an editing team that’s reviewing and cleaning up that translation to make it speak in the natural language.
Additionally, for machine translation, you can do both statistical machine translation that analyzes the language from multiple components and from the neural machine translation that we talked about earlier. These two technologies used together can allow for a much better translation capability.
And then finally, we talked a little bit about this before. But you can also accept enhanced machine translation. This means the ability to add the custom language dictionaries but also to allow machine translation with either light or full post-editing of the machine translation, which would allow that language translation to be more naturally written and give the reviewer the ability to understand the nature of the document.
So let’s talk a little bit about emerging data types. Emerging data types are data types that are coming into the forefront. Today, we’re going to talk about one specific one, which is Slack. But we also want to talk, point out that individuals are looking at all different emerging data types as they come about. For example, collaboration tools, such as Slack, are now becoming more commonplace than email.
So the ability to collect from them is becoming something that is absolutely necessary. But what about other emerging data types? What about home automation and voice control technologies such as Amazon, Alexa, or Google Assistant, or Microsoft’s Cortana or GPS and location tracking technologies? These new emerging data types are being reviewed and analyzed by eDiscovery tools as we speak, because individuals need to work with these technologies because they are becoming more commonplace.
With the GPS and location tracking technologies, there are tools out there today that allow individuals to determine where an individual has been, where they have been located, and what activity or tracking of that travel it is possible. As the emerging technologies come about, they’ll be able to do this in real-time and be able to analyze the data in relation to other documents or components, for example, on a mobile phone.
So let’s talk about one as a case study. Slack, which is a corporate collaboration component– and so for those of you that don’t know, Slack allows individuals to work together and collaborate by organizing messages in the channel, private direct messages, and multi-party messages. This would be similar to teams or other products.
The channels can be correlated to all types of things from teams, projects, parties, business locations, and other special business units. But while Slack allows for the corporate consumer to have a much easier time and be able to organize their data, the ease of the solution is what makes this data so complex to collect and analyze due to its extremely dynamic nature.
There are emerging technology tools, including Slack’s own discovery API that can be used by trained forensic experts and experienced information technology personnel to properly collect from Slack. It is very important to understand that Slack has many challenges. For example, much of the chat is small words and small phrases making, for example, technology-assisted review more difficult to view.
It also sets time zones and time and date. And conversations may or may not be related together even when they’re in different channels. So knowing how to look at Slack, identify what you need, and collect it from eDiscovery is an important step when dealing with Slack data.
Our forensic experts have been working with Slack for many years and have very good components that they look at when analyzing how collaboration tools should work. But they have to keep up with it because it is constantly changing. Even in the last month, concepts around Slack, Facebook, and other tools have already changed their way of looking at things and the way that they balance the eDiscovery component with the ease of use that they are asking of users.
The idea behind this is, is that emerging technologies are constantly changing. And what you need to do is think about how you’re going to approach this changing technology and look at it from a perspective of eDiscovery. With that, I want to turn this over to V to talk a little bit about review and how emerging technologies have changed that process.
Vazantha Meyers
Thank you, Todd. So the one thing I wanted to point out is that everything you talked about previously in terms of collection of data and processing data in its most usable form ends in [inaudible] and some of its more relevant data sets that are factors in making a review faster and allowing us to make faster decisions.
I did want to say, everything that you said earlier applies to review as well. It’s getting the data in it’s most useful forms, so the reviewers have less work to do when getting through the documents, therefore, saving costs and making better decisions.
So one of the technologies we’re going to talk about right now at AI and the forms that it shows up in terms of the review phase. But let’s just go over AI, in general. As most of you may know, artificial intelligence is technically the study of mental faculties through the use of computational models.
And what that means for the legal industry is that we are able to use AI to take human decisions and apply that through methods and methodologies to determine responsiveness, privilege, and issues on a automated level so the reviewer and people who are looking at documents are making decisions about that documents and to being fed into the computer. And the computer uses methodologies and models to then apply those decision making as best as can across multiple documents so the reviewer or human reviewers do not have to.
Technology-assisted review is the application of that methodology at the very beginning of a review or do bail-out phases of the review where reviewers are looking at documents and making coding decisions, on responsiveness, on privilege, on issue codes, on categorization of data essentially. And the computer then says to the human reviewer, I think I understand what you’re talking about. Let me apply decision-making that you’ve made during this phase to a lot of document through the rest of the documents that it has available.
And I’ll categorize the documents in a way that I think conforms to the way you’ve been coding the documents previously. What this does is it allows review team and anyone who is analyzing large sets of data to look at left data essentially. It’s a calling methodology. And it also can be a prioritization model.
It allows the reviewer to make coding decisions across a bunch of documents without having to look at each document through the use of AI. It’s really effective in calling out nonresponsive documents or irrelevant documents. A lot of what Todd talked about earlier does that for us in part. And then once we get to the review phase, technology can take that even a step further.
So we went over a little bit about technology-assisted review. And one thing that we like to point out is that technology-assisted review. And whatever platform it turns that to is, like it’s not to TAR 1.0. It’s not a different tool from TAR 2.0 or TAR 3.0. It’s really versions of the same solution.
The workflows change, and the methodologies changed but uses, at its core, a base categorization model. However, it’s constantly changing. What we found is we went very quickly from TAR 1.0 to TAR 2.0. And now we’re looking at TAR 3.0. And we’re also looking at ways to use AI to categorize data for the purposes of PHI identification or GDPR reporting or privacy rules compliance.
So we talked a little bit about the training phase for TAR 1.0. And the idea is that you start with all of the documents that you want to make analytical decision on. And you start with a control set and a sample set of those documents and just have reviewer review those documents and code those documents according to the analytical decisions that they are been asked to make.
The control set is random. And the decisions on those random documents are then applied to the remaining documents. For instance, if I have a review set of 200,000 documents I start, might start I’m looking at 1,000 documents and making coding decisions on those documents on the interface. And the computer then applies those coding decisions and says to the human reviewer, I’ve categorized the rest of those documents based on your coding decisions.
And what that then does is say, hey, reviewer, you don’t have to look at 50,000 of these documents because we think that they’re not responsive. And we also think that another 30,000 documents are highly responsive. And I’m comfortable with that decision. And then the reviewer might have to only look at half of that data set. We’ve even seen reductions of 70% using TAR 1.0.
Now, TAR 2.0 takes that a little further. So one of the fallbacks of TAR 1.0 is that you have to have your whole data set. And you’re making decisions on 1,000 documents at the very beginning of the review. As many of you know, your understanding of documents and the changes over the course of a review or a project, an investigation or litigation.
And so there’s some disadvantage of having to do all of this upfront– one being your decision-making will change over the course of a review. And you might actually be getting documents on a [INAUDIBLE] basis. So you might not have all of your documents. And TAR 2.0 answers that question. What it does is it applies the same methodology but as the reviewers are looking at the documents.
And so one, you don’t have to have the whole data set in order to start making coding decisions. And then as you’re making coding decisions, the computer is constantly updating its understanding of the decisions around those documents and applying that in real-time until it gets to the point that it says, hey, you’ve been reviewing these documents for a while. I’ve been sending you documents. I think a match– what you’re looking for– we’ve run out of documents.
And then at that point, a team can make a decision on whether or not they want to continue reviewing those documents or not. But as you can see, it’s the same methodology. It’s just applied in a different work model– one that is going to be advantageous in some review. And we found it to be very useful, even when we’re working with small sets of data.
So TAR 3.0– what does that? So it’s still requiring the same amount of as the same base algorithm. And it’s narrowly-focused clusters of six bags and concept space, meaning it applies 2.0 methodology, just the cluster document, which then ensures that a diverse set of potentially relevant documents are reviewed. Once no more relevant clusters can be found, the new review questions are used to then train the set. So it’s a combination of TAR 2.0 and TAR 3.0.
There’s still no need for control set. The system is well-trained, grew out via the regular traditional review methodologies. But then it can be applied at this TAR 1.0 throughout the data set, meaning now, we have a new way of working faster. We can get started. But we can also apply it in large performance apart as opposed to the continuous active form.
Before we go to questions and answers, I did want to point out one of the ways that AI is being used in a big way that results in a lot, we think, in a huge cost savings to clients. And we’ve been looking a lot of technologies that are coming out doing auto redaction, that auto-identification.
A large part of an investigation or even like reporting and compliance requirements now is the identification of data for the purposes of putting together reports of PII or redacting personal information or just identify potentially privileged and confidential information. And we found that in some cases, the computer can do that better and faster than the human reviewer.
We’ve used Blackout for years. And we’ve looked at, for instance, DocuVision AI has come out with a software program that has already been set, meaning we’ve taken some of the technology that we’ve discussed now in terms of teaching the computer how to identify documents. And it’s already been said with data format and information where it thinks that it can make a decision on what is confidential, what is privilege, and what is PI and PHI.
The way that’s applied for review and making it faster is that, one, when we have to make sure we redact PII, the computer can help an attorney or a reviewer or a paralegal identify that the instances of that PII, PHI in document. So it makes it faster to go through them. It can also autoredact at some level– some of that information, for instance.
If I know that there is a particular client name and a set of documents, and I need to redact that client’s name, the tool can go through an autoredact. A human reviewer can come back and edit it and look it over. But the computer is taking a lot of that work off of the reviewers, making the system better and faster and decision-making easier on those documents.
It’s also helpful when compiling reports. There’s been an uptick in data breach project and reporting requirements because of privacy laws across the world. And then the technology can go in and start grabbing that information and put it in a report form faster and easier than a human can do it. And so we’ve seen that, as the technology that we’re using now, changes and emerges we’re also applying it differently.
But at the end of the day, the takeaway is that it is allowing us to make better decisions on documents that we collect, better decisions on how we process documents, and what form we post those documents or make those usable and also how we go through and analyze and create data set based on review protocols and investigation requirement.
So I don’t know if we have any questions on anything that we’ve talked about. But I will turn it over to Rob if there are none.
Closing
Thank you, Vazantha. And we’ll continue to monitor the questions from the audience. But more than anything, thank you, Todd and Ashish for your excellent information and insight today. And I also want to thank everybody who took the time out of your busy schedule to attend today’s webcast. We know how valuable your time is. And we appreciate you sharing it with us today.
Also, I hope you have an opportunity to attend our next monthly webcast. And it’s scheduled for June 17 at 12:00 PM Eastern time. And it’s going to be on the topic of remote discovery. And in this presentation, it’ll be hosted by the Electronic Discovery Reference Model or EDRM.
We’ll have a team of eDiscovery technologists and authorities share remote eDiscovery inside, specifically in the areas of collection and in review. And we’ll also have a detailed demonstration of how HaystackID’s remote offerings may benefit eDiscovery professionals as they consider eDiscovery in our new remote world.
As we have no questions and being cognizant of your time, I’ll just go ahead and close out now, and thank you again for attending and wish you a safe, healthy, and great week from HaystackID ID. Have a great day. Thank you. That concludes our presentation.
CLICK HERE TO DOWNLOAD THE PRESENTATION SLIDES
The Only Thing Constant is Change – HaystackID – 052020- FinalCLICK HERE FOR THE ON-DEMAND PRESENTATION (BrightTalk)