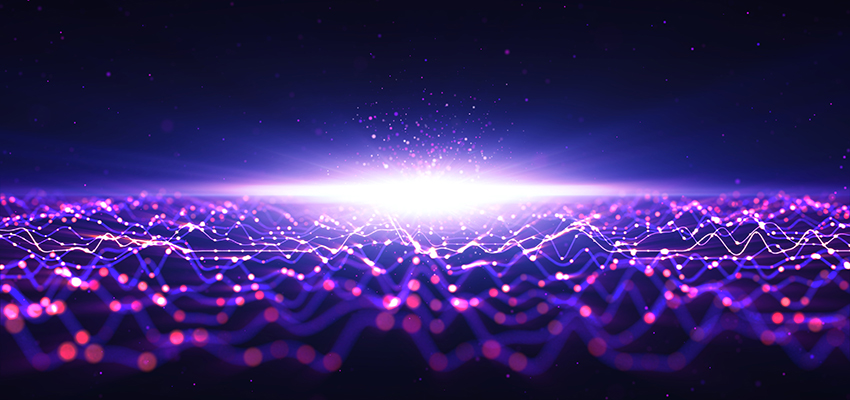
[Webcast Transcript] Demystifying Artificial Intelligence, ChatGPT, and Large Language Models in the Legal Industry
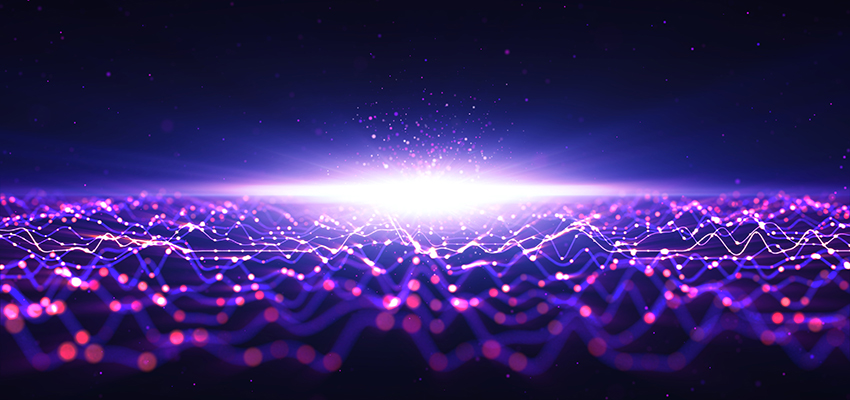
Editor’s Note: As the legal industry stands on the cusp of a technological revolution, the application of artificial intelligence presents a new frontier for legal professionals. Our recent webcast, “Demystifying Artificial Intelligence, ChatGPT, and Large Language Models in the Legal Industry,” held on October 25, 2023, ventured into the heart of this burgeoning domain.
The dialogue within this webcast was designed to illuminate the intricacies of AI technologies that are rapidly reshaping legal processes. In an era where the mention of AI is ubiquitous in legal discourse, there remains a substantial knowledge gap among practitioners regarding the practical impact of generative AI and large language models like ChatGPT on the legal profession.
This transcript offers a window into expert discussions on how these technologies can be harnessed to not only enhance the quality, efficiency, and cost-effectiveness of legal services but also adhere to the ethical standards that govern our profession. The explored use cases range from contract drafting and management to the sophisticated application of AI in depositions, concept clustering, social network analysis, and litigation research.
For legal departments and law firms seeking to navigate the intersection of technology and law, this transcript is an essential guide to understanding and integrating AI into their workflows. It stands as a testament to our commitment to advancing legal practice through innovation while maintaining the highest ethical benchmarks.
Download the presentation PDF and follow along with the rich insights provided in this webcast transcript; we are confident that it will both inform and inspire your approach to the integration of artificial intelligence in the legal field.
[Webcast Transcript] Demystifying Artificial Intelligence, ChatGPT, and Large Language Models in the Legal Industry
Presenting Experts
+ John Rosenthal
Partner and Chair of eDiscovery & Information Governance Practice, Winston & Strawn LLP
+ Ashish Prasad
Vice President and General Counsel, HaystackID
Presentation Transcript*
Support Moderator
Hello everyone, and welcome to today’s webinar. We have a great session lined up for you today. Before we get started, there are just a few general housekeeping points to cover. First and foremost, please use the online question tool to post any questions that you have, and we will share them with our speakers. Second, if you experience any technical difficulties today, please use the same question tool, and a member of our admin team will be on hand to support you. And finally, just to note, the session is being recorded, and we’ll be sharing a copy of the recording with you via email in the coming days. So, without further ado, I’d like to hand it over to our speakers to get us started.
Ashish Prasad
Thank you very much. Good afternoon, everyone, and welcome to the latest installment of the HaystackID webinar series. Today, we’re going to be talking about Demystifying Artificial Intelligence, ChatGPT, and Large Language Models in the Legal Industry. My name is Ashish Prasad. I’m the Vice President and General Counsel of HaystackID, and it’s my pleasure to serve as the moderator for our session. We have a special treat today in that we have as our speaker one of the leading lawyers on the topic of litigation, technology, and eDiscovery in the country. And that is John Rosenthal from Winston & Strawn. John is a litigation partner at Winston & Strawn, where he handles complex antitrust and other types of litigation. He is also the chair of the electronic discovery and information governance practice at the firm.
John has a 25-year track record of being one of the legal industry’s experts on litigation technology as well as electronic discovery. He is Chambers ranked, he’s the editor of multiple treatises in these areas, and the author of dozens of publications and hundreds of legal education seminars on these topics over the past 25 years. Why don’t you join me in welcoming him virtually, and then we’re going to ask him to walk us through the new world of artificial intelligence, machine learning, and related topics in the legal industry and guide us on how we ought to be thinking about these issues. John, thank you so much for being here. We’re really appreciative of your giving your time to educate us on these topics.
John Rosenthal
Great, thank you, Ashish. Could we go to the, and apologize to the viewers. We were having a little technical difficulty and can’t get out of presentation mode. Hopefully, you can read the slides. What I’d like to do is give you a little background about some of the latest AI technology, most of what you hear at the buzzword ChatGPT, ChatGPT-3, four, Bard, and platforms like that. Talk about its application in the legal industry. Talk just briefly about some of the ethical issues around its use, give you some best practices regarding adoption, and then answer any questions you may have in the chat. Please, if you do have questions, just put them in the chat, and we’ll try and answer them. So Ashish, if we can go to the next slide.
So, part of this is hype and buzz. The reality is that artificial intelligence really has its origins in the 1950s, and some form of artificial intelligence, be it Bayesian classifiers or other machine-based learning, has actually been around in the legal industry for quite some time. And for those of us that have been involved in eDiscovery, it’s been involved in eDiscovery for a significant period of time, at least the last ten years. And most of us are familiar with things like searching TAR classifiers, concept and clustering models, visual models that help you map out communications patterns and those kinds of things. Those all have a form of artificial intelligence behind them, but it’s very different than the latest form of artificial intelligence. So let’s go to the next slide.
So while these newer form of classifiers actually been around for a few years, it’s really in March of 2023 that the splash hit, and the splash basically hit because of the introduction of ChatGPT-3, which we’re going to go in a little more detail on what it is and how it functions. But there has been dozens and dozens of articles. You can hardly read a newspaper magazine or an article online without seeing some kind of headline about the transformational change. What’s transformational change? It’s change that you really can’t see or you can’t see its necessarily impact unfold for your eyes. Think about things like the introduction of the personal computer. People necessarily couldn’t grasp the transformational change that would happen in business or the practice of law at that time.
Another example is the introduction of the Blackberry or the smartphone. People could not imagine that the way that we do our daily work and lives will transformationally change. And that’s what we’re talking about here. So if we can go to the next slide. We see a lot of splash, and we’ve seen a lot of articles starting in around March. And by way of examples, here’s where a ChatGPT-3 engine passed the bar exam. And that started the immediate, our jobs are over, will computers replace us? What’s the future in the legal industry? And what I would’ve to say is like the introduction of any transformational technology, you have hope, you have fear, you have curiosity, you have hype. And think about it as the gold rush, right? You have something that has a lot of potential. You have a lot of people rush in with a lot of hype. There’s a lot of general understanding. You have people overplaying what it does, what it doesn’t do, what its potential is.
And that’s really where we are right now. These models have tremendous practical implications in the legal industry as well as almost every industry. And it will transform over time how we work, how many lawyers it takes to do certain tasks, how long it takes to do certain tasks. Is it going to replace lawyers? No. Maybe you need a different type of lawyer over time and one that’s a little more savvy in terms of working in these models. Maybe you need less of them, but it’s not going to ultimately replace lawyers. It really is how do we adapt the tool to the people in the processes. So, let’s go to the next slide here.
For us geeks that like animated movies or movies of action heroes, with great power comes great responsibility. You would not give a child a loaded gun. So we can’t give a lawyer a loaded ChatGPT-3 engine without giving them some guardrails, a policy, and some training. And what you’re seeing in some of the newspapers is hype around that. You’ve seen one lawyer who basically let one of these engines draft its brief, and it made up six cases, right? And when the judge said, did you take any steps to validate that the cases that the engine was generating in your brief were accurate? And he said, yes, your honor. I asked the engine, are these cases real? And the engine said yes, but of course, they were made up. You have a recent case last week came out where an attorney let a ChatGPT-3 engine write its closing argument.
And sure enough, the closing argument didn’t look anything like the actual trial. So there are potential dangers here, and we’re going to talk about dangers and best practices. So let’s go to the next slide. Now, I would say that there’s been a lot of immediate judicial reaction to particularly these incidences I just talked about, and you have a few judges and a few courts that have issued standing orders that say, thou shall disclose the use of any generative AI engine in your brief submissions, et cetera. I frankly think that these are really knee-jerk reactions and I think there’s a real danger to these knee-jerk reactions, right? Generally, the law, local rules lag technology. If we try and adapt laws and guidelines and procedures at the front end of technology when we don’t even know how it works, there’s going to be a real, real danger of inhibiting the technology.
Paul Graham and Marc Grossman have already written on this topic, and they wrote in a recent publication in October, while the impulse underlying the imposition of technology orders is understandable, even commendable, there’s real disadvantages in doing so. For example, some orders have been overly broad-sweeping in their scope, AI applications that do not produce final work product and do not suffer from generative AI’s propensity to hallucinate. Such orders may infringe attorney work product and discourage the use of the technology that will over time increase the justice for unrepresented litigants and reduce costs.
But with that said, I’m not sure we can necessarily stop these courts, but caution everyone is going to have to pay attention to how courts and judges issue standing orders and local rules regarding the use of these things. So let’s turn to the next slide. Go ahead.
Ashish Prasad
Before you do that, I was wondering if you don’t mind if I ask a follow-up question about that?
John Rosenthal
You may ask anything you’d like.
Ashish Prasad
Great. Let’s say that a practitioner today has a case, and he or she is going to use generative AI to prepare a draft of the pleadings. If there’s no court-mandated disclosure order in effect in that case, does the practitioner have an obligation to disclose that he or she used generative AI in preparing the brief or not?
John Rosenthal
No, I don’t think they have an obligation to disclose that. You wouldn’t have an obligation to the fact that you use LexisNexis or Westlaw to conduct your legal research or brief catch to do site checking and verification of authority. The real question is who’s validating. When you sign a Rule 26(g) certification to any pleading, you’re certifying the court that it’s done in good faith, that you’ve done your due diligence, right? Same thing with rule 11 when you draft a complaint or an answer, right? So the question is, are you doing the necessary homework to validate the results of the engine that you’re using for the purpose that you’re using it for? And we’re going to talk a little about what that means. But I would say absent a standing order or a local rule, I don’t think there’s an obligation to come forward and disclose.
Ashish Prasad
Okay, thank you John. That’s super helpful.
John Rosenthal
All right, so let’s get into the little geek speak because I think that we have to understand what these engines are just a little, right? So, apologies, and I’m not trying to denigrate the audience, but this is really AI for dummies, right? I consider myself a dummy and this is the way I really think about these things. So first of all, this is all about math. We all went to law school to avoid math, but we’re stuck back in math. The engineers have got us. This is about probability patterns, context meaning. I’m going to get to those where that fits in. But it really starts with machine learning. And machine learning is really just a branch of artificial intelligence that focuses on teaching machines to recognize patterns of data and make predictions or decisions based upon those patterns. It’s math, it’s pure math. That is what all machine learning models do.
Now, a variation of a machine learning model is a large language model, and it’s where an algorithm, a formula, is trained on a large amount of text-based data, typically taken from the internet and covers webpages, any kind of source you can do. So you take that same algorithm, that machine learning algorithm, and you train it across a large amount of text base. Now, there are large language models that actually operate across video, images, visual arts, and as you see these engines expand, you’re going to see not just text-based chat systems, but things like Dall-E that actually generate graphics and videos. But these are all large language models and you’re also going to hear about foundational large language models because there are a handful of very big ones that everyone is using as the base model from which to create a chat-based generative AI model.
Now, a variation of a large language model is generative AI. And the difference here is, think about when you run a Google search, a Google search searches the internet and it pulls back a list of hits. It’s not generating any information, it’s just saying, this information is located here, here, and here. The difference is generative AI generates outputs based upon patterns of data which they’ve been trained. It’s actually generating results. It’s not pulling back existing results, it’s generating text, it’s generating a piece of correspondence, it’s generating a legal memo. Now the game changer is natural language processing, because if we pile on top a generated AI engine, which have actually been around for five or more years, so what? Right? Because you need a computer scientist to use that or somebody in your IT department.
But if you add a chatbot to it, the ability to actually ask questions and get answers and interact like a human, that’s the game changer. And ChatGPT-3, which exploded in March of 2003, the big difference between that and the prior versions of ChatGPT is it added this chatbot. It allowed us dummies to say, tell us what the standard for the Ninth Circuit is for summary judgment. And boom, you get an answer. That caused the explosion because all of a sudden this technology isn’t necessarily limited to IT or computer scientists, it gives everybody access to this for a whole variety of applications. And that is the transformational changes. Now I’m going to quickly run through the next couple of slides just to give you a little more about, well, in terms of the models, again, you have these big foundational models like ChatGPT, Bard, Bing and there’s a spectrum. And these models are changed.
These are what’s called general-purpose models trained across broad swaths of information, essentially almost the whole internet. And they’re typically trained up to a point in time. I think ChatGPT-3 is trained up until 2001, although it may have been supplemented recently. And then you have these more specific models like the Google, Microsoft Copilot that people are developing. And then you have even more specific bespoke or industry models that are really not just trained general purposely, but they’re trained on a specific topic. So for example, in our space, you’re getting legal specific trained model. They’re using those foundational models as a start, but then they’re adding specific training to a specific industry or a task in order to refine the output of the model.
Now, let’s just quickly using ChatGPT-3, if we could go to the next slide. Talk about really how do these models work? How do you get here? And again, this is all probability and math. So we’re going to use the ChatGPT-3 as an example. And if we can go to the next slide. If we can go to the next slide.
There you go. All right, so let’s think about, this is about probability. What these engines do is, based upon their training, and I’ll come back to training, these engines, the ChatGPT-3 and just so you know, GPT transfer generative meaning it generates words, it’s pre-trained, it uses training supervised and unsupervised to allow you to make a query and respond to it. And transformer is the underlying technology, some of which we’ve already talked about. Now, if we talk about how this specifically works, and I would urge you to look at Dr. Rama Ramakrishnan, he’s a Professor of Practice at MIT Sloan School of Management, and I think that he has some of the best educational materials around on how this modern AI works. But-
Ashish Prasad
Sorry, John, can you give that name again for the audience please?
John Rosenthal
Dr. Ramakrishnan, R-A-M-A-K-R-I-S-H-A-I-N, Professor of Practice at MIT Sloan School of Management. He really is absolutely brilliant and he’s able to distill these things into very simple concepts. So what we have here is a large language model with a chatbot where if you put an input in, you make what’s called a prompt and you ask it a question, it gives you an answer. So if you say, and it’s all based upon probability, and what it’s doing is it’s predicting words, right? Every time you make a query, it predicts a word and it’s predicting the next word. So let’s go to the next slide. So for example, I have four score and I put it as an input, right? It’s based upon its training, it’s going through and looking at different potential answers.
And based upon its training, in the back of its mind it’s coming up with a probability ranking of what the next potential word is. And in this case, based upon his training, the probability is that the next word is seven. So let’s go to the next slide. It’s selecting that as the answer to come back to you. Let’s go to the next slide. And what it’s going to do is iterative. So if you go, tell me and finish the sentence four score, and it’s going to go through this and it’s going to go multiple generations, and it’s going to predict word and word and next word. So let’s go to the next slide. So as you can see, it’s going to give a probability that this is the most next likely word and the most next likely word, and then it’ll build a sentence and then based upon its training it will build an entire sentence or paragraph. Next slide.
So imagine based upon a prompt a question, it’s doing this not ten times, not 100 times, but it’s 1,000 times. It’s constantly accessing its training to respond in a conversational style and predict the next word or series of words or sentence or paragraph that’s responsive. Now, a couple important things about this, just so you understand this. So how does it get here? Because this is not just word response. It’s not trained to always say, if you use this word, here’s the response. It’s not pattern-matching with exact matches. It’s using two kinds of training. The first is supervised human training. So at the beginning when they started with the foundational model, they actually asked questions, a human asked questions, and then it gave feedback, because sometimes you could have the same answer or different answers to the same question. So not only did it give multiple answers, but the humans also ranked the answers.
So it’s not always that you would get the same answer. Again, this is all praised upon probability, but you can also in these engines ask the same questions and get a different result, because there’s also a randomizer built into some of these foundational models. So human learning can only take it so far, right? Because it can only have so many humans, and that’s for example, what ChatGPT-3 did, OpenAI trained it using humans across almost all of the internet. But human training can only do so much. You can have only so many human do it. So there’s a second part of the training, unsupervised human, where they actually used algorithms to simulate the human training, the feedback loop, and the ranking, to then train the model across thousands and hundreds of thousands of question-answer scenarios.
So that’s one of the advantages here, that it’s not just trained on 1,000 or 100,000, it’s trained on millions and millions of questions and answers where it has the ability to rank the probability. So again, you have public models where you’re training it against the world, foundational models, and then you have these specific industry models where you’re then taking it and refining the training more, for example, across legal concepts. So let’s go to the next slide. So what does this have the potential? Has the potential to answer questions, to do text classifications, to summarize documents, to generate text such as letters, briefs, et cetera. Let’s go to the next slide.
So let me give you a practical example. If you don’t have it, you will have it. There’s a premium service that’s coming to Microsoft Teams, right? So in your virtual meeting, it will generate a verbatim transcript of your meeting. So that’s not modern AI. That’s basically the ability to do text to speech conversion. But what this new service will do, it will run the entire transcript through a generative AI model, and it will develop notes and action points coming out of your meeting. The generative AI model will actually tell you, here’s the meeting, here’s the highlights of the meeting. Here are the action points that we think that the generative AI engine thinks should come out of the meeting. That’s a practical example of how transformative this will be, at least in the general business setting. Now, let’s go to the next slide.
There’s some problems with generative AI. It gets things wrong. It’s all a question of the quality of the training, but it doesn’t have built-in guardrails. It doesn’t. So for the most part, when you have a model, you need to look at whether or not there are guardrails built in. So for a lot of these industry-specific models that people are building, they’re building in not only an industry-specific engine that’s trained on an industry-specific issue such as legal research, but they’re building guardrails, the ability to check against, for example, a real set of known database of cases like the LexisNexis database, to figure out whether or not it’s getting things wrong. The same thing is it makes up things, just like it made up those six cases that the attorney asked the engine to do. And when it wrote a brief, it will make up things called hallucinations.
And again, do you have guardrails built in some of these engines to prevent hallucinations? Sometimes you do, sometimes you don’t. Which goes to the issue of validation, right? You have to validate that the results aren’t made up or wrong. Next, it can’t understand motion and empathy like a human. One, they’re built in biases, right? There’s data bias, style bias, historical bias, user bias. You have to be careful about that, particularly if you’re going to use the engine to evaluate, for example, candidates that are applying for a job, right? There are a lot of open legal questions around it because it scraped the internet in order to do the training. Is that copyright violation? Privacy, again, scraped the internet and is scraping databases, which includes individuals’ PII. Do we have privacy violations?
Defamation. There have been cases where people have published something generated by a generative AI engine and they’ve been sued for defamation. There’s going to be a whole host of things about if a generative AI engine writes an analysis or a critique of somebody’s performance, does that rise to the level of false advertising, defamation, et cetera? I would say there are a lot of hopes, but there are also some issues with it. So let’s go to the next slide.
So let’s talk about legal uses, right? Next slide. So there is no question in the legal industry that this is going to have dramatic impact. Some of the general-purpose engines have application now. There are several providers with industry-specific engines. And over time there are only going to be more engines and the engines are going to get better. I would say not all are created equal, but in terms of things that some of these things can do now, they can generate correspondence, they could generate a timeline of events, they could review documents and tell you whether the document is potentially relevant or privileged. It could draft correspondence, it could draft document requests, it could draft interrogatories, it could answer a complaint, it could draft a policy. It could look at transactional documents and say, okay, this is how it’s suggest improving the transactional documents.
Pretty much any question you could ask it it can give you a response. You could also upload documents and say, look at this document, tell me how to improve it. Or for example, I loaded 144-page complaint in a public document in one engine to test it. I said, give me a timeline. Identify the key players, identify all the significant documents, and it pretty much gave me a work product. It had to be cleaned up. But had I given that to an associate, it probably would’ve taken three hours of work to do. It literally took 10 minutes to get something that was, I would say, okay. So in order to give you a little practicality here, what I really want to do is quickly just show you. Next slide. One of these engines in practice. One of the legal-specific engines is CoCounsel by Casetext.
Casetext was just bought by Thomson Reuters. Thomson Reuters has incorporated into their offering. You have similar offerings out there from others. So this is just the one that I have used and we got in on the ground floor with this provider and helped them bring their engine along. I just wanted to show you that this is not vaporware, this exists today. Let’s go to the next slide. Very simple interface. I apologize if you can’t see it, but at the bottom it basically it’s got a line, and like Google, you say, tell me X or What’s this or give me the standard again in the District of Columbia for false advertising. So let’s go to the next slide. And I know this is hard to read, but this is an example of some of the skills and they’ve been building in skills over time. It can do legal research, it can summarize documents, it can generate correspondence, it can help you prepare for a deposition. It can analyze documents.
Let me give you a couple of practical examples. Next slide. So again, I apologize and we can distribute these slides after they’re difficult to read in the presentation mode. But research memos, I’ve been using it now for about six months to do basic research memos. Now, I would tell you over time it has proved as they continue to train the model and they’ve trained it over legal-specific data sets. And often the quality of the results depends upon what you call the prompt or the question, right? So if you say, give me a simple, if you’re not very detailed or accurate on your prompt, you’ll probably get a very simple, not detailed response, if you’re more specific. But this particular engine also allows you to iterate. So you can look at the results and say, okay, we’ll take those results and now do this.
And I would say, is it going to give me a perfect research memo? Probably not. Is it going to give me a big leg up and reduce the amount of basic time that I have to do to get a basic framework in place? Yes. Now, in terms of validation, this is an example. They’ve built-in guardrails against a real database of real cases, right? So they’re behind the scenes validating the results of the engine against a real set of cases. If you went out on a general ChatGPT engine or use something like Bard or Bing and said, give me a legal research memo on X, there’s probably no guardrail built into it, which is why that one attorney when he used a general purpose engine to draft a brief, ended up with hallucinations. Next slide.
So again, you have the ability to upload and analyze documents. And I know this one is very hard to read, but this I thought was interesting because you uploaded a document that was an email, simulated email, and it says, an attorney on law and order is so handsome. I would love to see his work product. I would love to have privileged communications with him. These communications would be confidential, if you know what I mean. So load that into the engine and say, is it privileged? And it comes back and says, no, it’s not privileged. It doesn’t reflect the request for or provision of legal advice. It tells you where these engines are heading, particularly in the eDiscovery space and the ability to analyze documents. Next slide.
So again, I think I already went over the document summary, but you can upload documents and different of these engines have limitations on how many you can upload. But for example, Casetext says they can upload on the order of a million documents for analysis. Next slide. So what we’re seeing is a rush of providers and startups coming in to offer these in the legal industry for legal research, for brief writing, for transactional work, for due diligence analysis. And what you’re going to see is the classic cycle where lots of people rush in and over time the product will improve, the providers will consolidate and you’ll have a handful of survivors, I would say five to 10 years from now. Can these engines add value now? Yes.
But let me talk about, for example, in the eDiscovery space, we have Relativity, we have DISCO, we have Everlaw, they’re all rushing to put this kind of functionality into their eDiscovery suite and will be released soon. But the real question is, what’s the quality of these and what kind of security that is wrapped around it so that you can do this in a way that will not jeopardize privileged and confidential information? I’ve seen and beta tested these engines. They definitely are going to improve the quality of what we do, particularly in eDiscovery, but I do think it’s going to take a little while, probably a year to really shake out how well these tools work as well as processes and procedures around their use. Next slide.
Ashish Prasad
John, if I could just ask two questions at this point. First, do you foresee a time when contract attorneys who are today reviewing documents for relevance, privilege and so on are replaced by generative AI and LLMs? Or do you think that the generative AI and LLMs will over time be used to help those contract attorneys make faster and more accurate judgements but not actually replace them?
John Rosenthal
Okay, so I think, and I get this question all the time, and I think this applies to all attorneys, it will help you do your job better so you become more productive and more accurate, no question about it. And that’s true in the review setting, particularly in the more complex things when we’re looking at such as privilege. It will also give you added capabilities because you’ll able to take these engines and somebody well-trained on it will be able to go to the next level and not only review them for confidentiality privilege, but you’ll be able to identify them for witness kits, trial kits, fact modules. It’ll give you the ability to become more integrated with your case team and provide more services. I think over time you will need less, right?
I don’t think it’ll replace the job. I actually think the job becomes more important, because lawyers are going to look for people with a particularly skilled on how to use these engines to become more valuable members of the case team. But I do think over time you’ll need less to do the same. Now, with that said, the amount of eDiscovery that continues to go in the pipeline continues to grow. So the question is, does it allow us just to do more eDiscovery or are we going to be proportional and do the same amount with less people? That I can’t predict. If I could, I wouldn’t be practicing law. All right. Great question.
Ashish Prasad
Okay, great. Thank you. Thank you, John. The second question that I want to ask you has to do with judges. It seems to me from your remarks that the LLM capabilities are there today to provide a judge who has a case with the first draft of an opinion in that case based on past opinions with similar fact patterns. Do I have that right? And if I do have that right, what are the implications for the administration of justice if judges can get an AI-generated draft of their opinions?
John Rosenthal
I don’t think there’s a downside to a judge doing the same thing an associate would do, be getting an AI-generated draft of a brief. It’s a question of whether they’re doing independent research, independent validation. And also the judge is supposed to be doing independent thinking, right? It’s okay to use a tool to help refine your thinking, but at the end of the day you can’t do what some of these practitioners are doing, which is just having it generated, sending it out the door without validating and doing some independent work.
Ashish Prasad
Got it. Okay. Thank you, John.
John Rosenthal
I’m very quickly going to talk about some of the ethical rules. There are lots of ethical rules and almost the ABA has formed a task force to look at this and its implications. Various state bars from California to Florida either have produced some initial guidance or looking at what guidance to provide or whether their state rules need further refinement. There are lots of rules that are implicated. Rule 1.1 on competence, 1.2 on scope of representation, 1.3 on diligence, 1.4 on communications, 1.5 on fees, 1.6 on confidentiality, 3.3 on Canada Tribunal, 5.1 responsibilities of the partner to supervise the lawyer, 8.4 on misconduct as it relates to not doing something that would bias or discriminate against somebody. So lots of implications about the ethical adoption and use of these. I’m going to focus on the couple big ones.
So the first one is competence. Under 1.1, you have a duty of competence. So that means it’s incumbent upon our attorneys if we’re going to use it to understand it. And if we are not going to become the subject matter expert, have somebody on your staff or a consultant that is a subject matter expert. I would think the first thing you really have to do as a practitioner or as a large firm or as a corporate department, is really exercise your duty of competence. Figure out who your subject matter expert’s going to be and focus on a program around this. I’ll get to a little more what that looks like. Next slide. You have a duty to exercise independent professional judgment and render candid advice, going to what she’s just mentioned.
That doesn’t mean pressing a button, letting the machine generate the advice. You have a duty to do independent work here, right? Let’s keep that in mind. Next slide. You have a duty not to reveal your client’s competencies. And I’m going to spend the most part on this because I think this is the most complex issue here. So if I’m asking, uploading. Let’s go to the next slide, because I think this will help. And state bars have already kind of addressed a little of this because there are many state bar opinions that had said it is okay to use cloud services. It’s okay, you no longer have to keep it in a filing cabinet or in a data center that you run or a server you own. It’s okay to use cloud services, provided you’ve taken reasonable steps to protect the confidential information.
Because what we want to do is keep our clients trade secrets. We also have an obligation to keep confidential our communications and work product. Otherwise, they’re his waiver. And the courts have already said with cloud computing, it’s okay. But those opinions are very superficial and they were early on and they don’t really identify what do they mean by cloud computing? Does that mean I got my servers co-located in somebody’s cloud or does it mean I’m using somebody’s cloud like Office 365? So it’s actually not my cloud, it’s a shared cloud and there might be logical separation between documents, but it’s all in a shared cloud. Or does it mean actually it’s not my cloud and it’s not my provider’s cloud, it’s in yet somebody else’s cloud that links into my provider where I don’t have privity of contract and I don’t even know what that third party cloud provider who’s providing services to the cloud provider that I am providing to what they’re doing with my data.
This is all going to have to unfold. And these are real issues that a lot of the providers are trying to gloss over, say, don’t worry, it’s secure. For example, OpenAI which owns ChatGPT-3 says it won’t save your documents, it will not use your documents for training, it will delete your documents after X number of days or upon request. Right? Now that’s great, but can we imagine how many queries OpenAI gets a day? Can we imagine how many lawsuits they’re currently in and whether they have a legal obligation to hold all those things despite what their representations are in their terms of use? Do I have a privy of contract with OpenAI? Probably not if you’re using one of these industry-specific providers who’s then using, has a contractual relationship with a provider that’s providing one of these foundational models. Next slide.
So this has already really happened. We’ve had several examples. Here’s a Samsung where somebody loaded up a trade secret document to one of these engines and it all of a sudden was in the public domain. So here’s a practical example. Let me just go through this quickly because I know we’re running out of time. Next slide. So this is typically how one of these engines might work, right? There’s a web app. You open up on your computer, you make your query, it goes to the provider. They have their container where they have their application and they store the documents you submit and they’re doing processing and they have their industry-specific model there. But to do the heavy lifting services, like drafting a document, summarizing a document, it actually reaches outside of their container and goes into OpenAI, right?
And then OpenAI feeds back the result and then they feed the result back to you, right? So maybe you have a contract with the provider, but do you have a contract with OpenAI? And while I tell the provider to keep my stuff confidential, if my provider sends the information to OpenAI, is that somehow waiving my privilege or putting my trade secrets at work? And a lot of people are glossing over this. Saying, what’s the big deal? You put your stuff up in the Microsoft cloud? Nobody questions these things. I think it is a potentially big deal, particularly since none of the state bars or the ABA hasn’t really talked about this. So next slide.
Now one one-step solution is kind of what Microsoft’s doing. Let’s put it all in our cloud and we’ll apply our own version of these generated AI tools where you are in privity of contract. And while there are lots of people in our cloud, it’s containerized to our cloud. Again, probably safer, but nobody’s really articulated and nobody has said, look, that’s okay and doesn’t put our confidentiality and privilege at risk. Next slide. So let’s just go to the next slide because we’re out of time. So will artificial intelligence replace lawyers? No. Next slide. But what are its implications? Certainly for law firm, staffing. It’s going to impact speed efficiency, technological expertise, staffing models. It may impact revenue and profitability because a client may say, if it would take an engine 10 minutes to draft a base memo for which then you would edit, why would I pay you the rate of a second or third year, five hours to do the same work?
Risk exposure, what we just talked about in terms of confidentiality privilege. On the client side, how are you going to let people use your confidential information? And while it’s going to lower the cost of routine tasks, it’s not without a risk if you don’t bring in the talent that knows how to use the engines and make sure the engines have the appropriate guardrails. Is it going to allow clients to potentially in-source more? Potentially, yes. When you have engagements with your law firms, you’re actually going to have to tell them whether or not they can use this technology and how they can use it, how they’re going to charge for it. On the business side, businesses are going to use these tools too. So in terms of advising and counseling, how do you protect your trade secrets? Who owns the data?
If you’re using a public model that’s scraping the internet, are you engaged in contrarily infringement? How do you use these things in conjunction with your customer and employee private data? How do you engage in contracting with vendors, suppliers, and customers regarding the use, adoption of these issues? These are all implications for the legal industry that everybody’s got to be focusing on. Here we’ve kind of formed a task force, and we are not only focusing these internally, but we’re focusing them in terms of how do we advise our clients. Next slide. I swear we’re almost done. Implications for litigation, they’re a lot, right? Because not only do you have these tools in your law firms, your opponents have them, right? If you’re a defense organization, particularly involved in asymmetrical litigation, these tools have a big advantage for your opposition.
They can do more with less, right? They can search across a million documents and say, tell me where all the documents are that reflect a design defect. Now, the quality of what they’re going to get now, probably pretty poor, over time it’s going to increase. Not only that, if you put these engines in on the business side, what’s going to prevent a request to inspect that, say, look, in your manufacturing system, I would like you to run the following query across your generative AI model, which is tell me where there’s been any exposure of a toxic chemical to an employee and describe that exposure, right? How do you prevent a request to inspect? Those issues are coming, and that’s something that we don’t have answers for but have to get ahead of as an industry. Next slide.
And I think this is the last slide. So what are your best practices around adoption? First of all, understand what you’re adopting. What’s the engine? Whatever its benefits, whatever its downsides. Does it have guardrails built in? You have to analyze the technology or bring in somebody that does. Understand the security risk around confidentiality and trade secret. You have to mitigate confidentiality risk either through policies or notices. You have to understand the quality of the results and the limitations of those results. You have to determine what guardrails to establish, right? For example, you have to put a policy forward that tells your associates and partners. You can’t just send out a brief out the door without doing independent validation of the contents of the brief, including the facts and the legal citations, and describe what you expect that validation to look like.
In addition to a policy, you need to have education and training. You just can’t give somebody a loaded weapon. You have to give them a policy about how to use that weapon and then educate them on that policy. And then you’re going to have to have some open communications between the law firms and the clients. Can you use it? Under what circumstances can you use it? How do you charge for it? We threw a great deal at you. Next slide. And I don’t know whether I can look and see whether we have any questions.
Ashish Prasad
I’ve looked through that, John. We’ve had a number of questions, but those questions came before you talked about the ethical rules, and you’ve already answered those questions. There’s one question though that has arisen that I’d like to get your take on, and then we’ll close out the webinar. We’ll go a couple of minutes extra because we started a little bit late due to technical problems. John, it seems pretty clear from your discussion of the ethical rules that we are going to see the state bar authorities amend the ethical rules to deal with generative AI, even if all they do is emphasize that generative AI must be used consistently with our duties as lawyers.
The question on my mind is whether there is scope in your mind for amendments to the federal rules of civil procedure to address use of generative AI in litigation as we have had amendments as you well know to address eDiscovery, do you see amendments coming to our civil rules to deal with generative AI or do you think our current rules as written can basically handle the use of generative AI in civil litigation?
John Rosenthal
So Ashish, you, like me, are a student of rules reform. I know we’ve worked together on a variety of rules reform over the last couple decades, including the adoption of the original eDiscovery rules. I testified in front of the rules committee last Monday on the new proposed privilege rules. I would say that in rules-based, the rules committee has always been very reticent to deal with technology. They want very general guidelines on technology. One, because they don’t want to inhibit the use of technology, but their also biggest fear is the rule will get leapt, right? Rules will move slower than technology. So if you put a rule in place and the technology transforms, so imagine if we put a rule in place on TAR five, six years ago.
First of all, it would’ve been put out in the context of TAR 1. Almost nobody uses TAR 1 today. So now we got TAR 2, right? And now we’re on the cusp of something where TAR will probably be obsolete in a few years, because people will be using some version of a generative AI engine. I think the ones now probably aren’t ready for full prime time. They’ll be beneficial. There’s a real danger. I think what we’re going to have to see is whether we see emerging issues and problems regarding its use. And then over time the rules committee will take up. I do think it’s going to have to look at issues particularly about requesting to inspect and provide some guidance over time. Because you’re going to have huge battles where, for example, can someone on the opposing side force you to use a generative AI engine to answer something or do something?
Because when you look at it, current discovery rules are based upon what is then in existence, right? Facts, information documents, then in existence. There’s no obligation to create anything in response to a document request. You can if you want to, but there’s no obligation, right? But you can imagine that people are going to say, well, you’ve got a generative AI engine. I want you to put the following prompt or query in there, right? So is that fair game? Because you’re not discovering then existed ESI, you’re asking somebody to generate ESI. I do think that issue is going to take some evidentiary and perhaps rules, but I think that it will be very slow. You’re looking at a multi-year horizon before I think there are federal rules that would even come close to addressing this.
Ashish Prasad
Excellent. Well, that’s, I think a very fitting way to end. Let me not just thank you, John, for giving us an hour and a half from your busy practice today, but let me also compliment you, your slides, and I’m sure the audience would agree, I can tell from the questions and comments I’m seeing, your slides are not only comprehensive but lucid. The area is complex, it’s new, it’s very confusing. And one gets the sense that a lot of people who are writing about this area have different kinds of motivations, which are maybe not lending themselves to the clearest presentation. So this was great. We really appreciate it. On behalf of HaystackID, I thank you. And I thank all of you in the audience for joining us. Our session is concluded, and everybody have a good and safe day. Thank you very much. Bye-Bye.
John Rosenthal
Great. Thank you Ashish, and thank you to HaystackID.
About HaystackID®
HaystackID is a specialized eDiscovery services firm that supports law firms and corporate legal departments and has increased its offerings and expanded with five acquisitions since 2018. Its core offerings now include Global Advisory, Discovery Intelligence, HaystackID Core™, and artificial intelligence-enhanced Global Managed Review services powered by ReviewRight®. The company has achieved ISO 27001 compliance and completed a SOC 2 Type 2 audit for all five trust principles for the third year in a row. Repeatedly recognized as a trusted service provider by prestigious publishers such as Chambers, Gartner, IDC, and The National Law Journal, HaystackID implements innovative cyber discovery services, enterprise solutions, and legal discovery offerings to leading companies across North America and Europe, all while providing best-in-class customer service and prioritizing security, privacy, and integrity. For more information about its suite of services, including programs and solutions for unique legal enterprise needs, please visit HaystackID.com.
Source: HaystackID
*Assisted by GAI and LLM technologies.