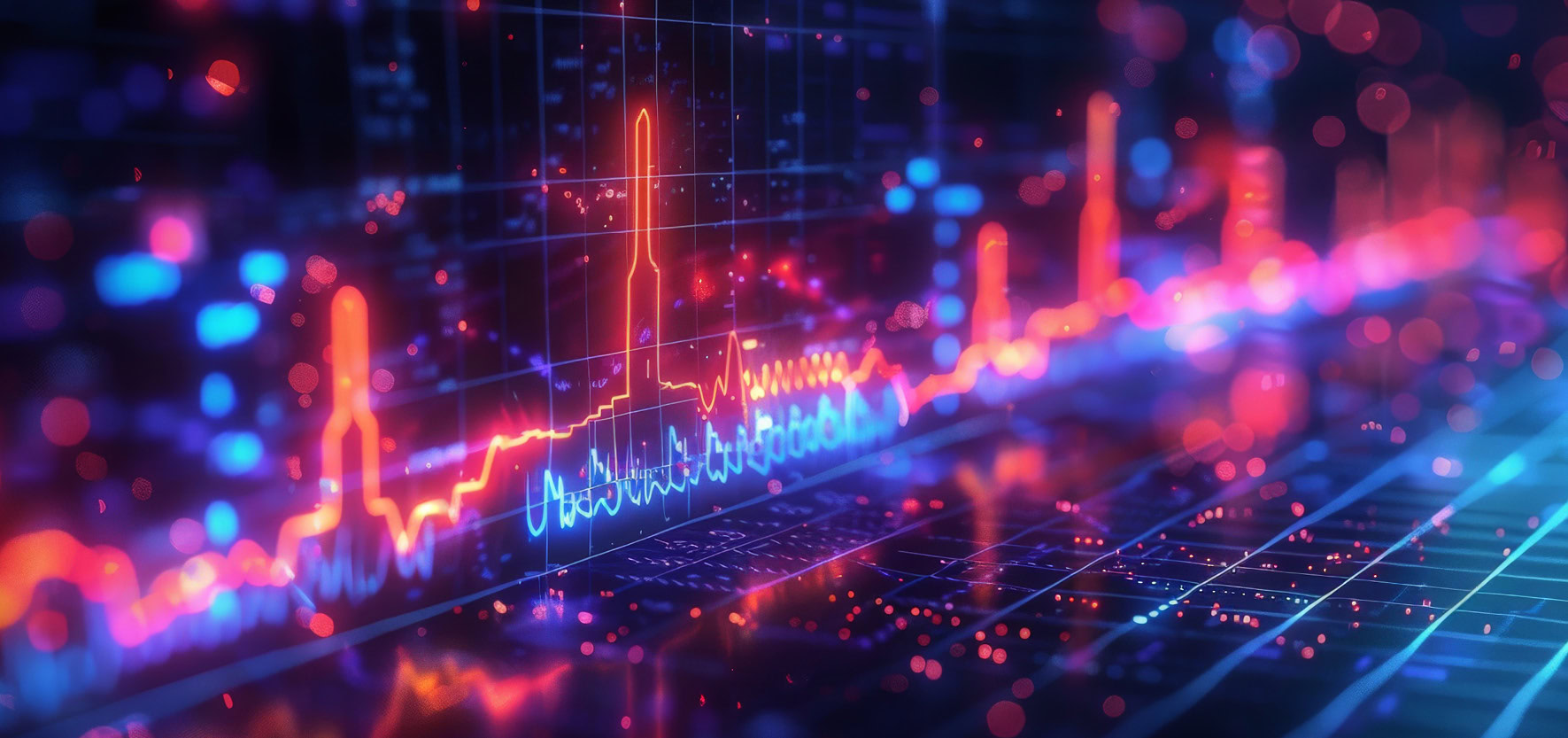
[Webcast Transcript] 6 AI Workflows to Enhance ESI Discovery
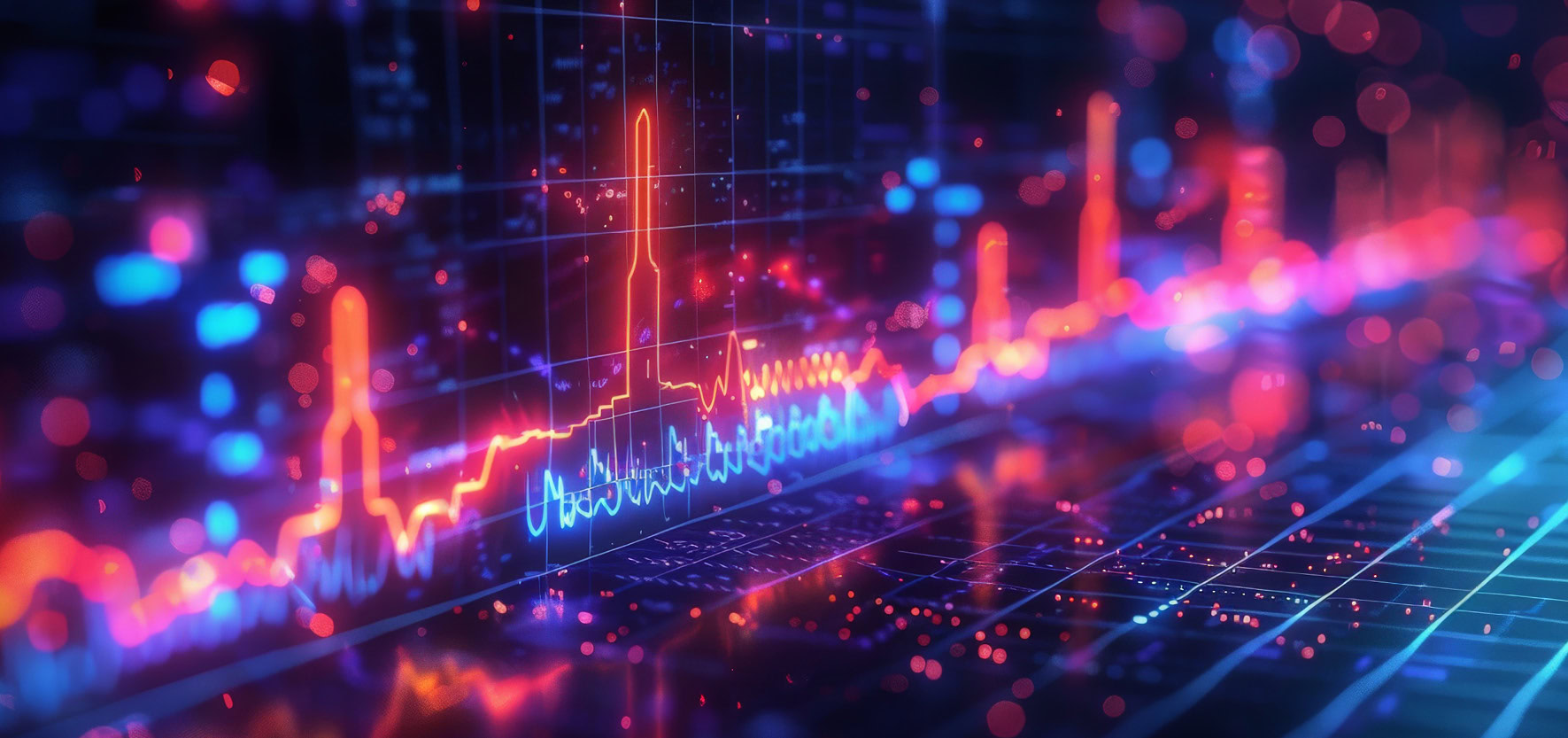
Editor’s Note: In a recent HaystackID webcast, legal professionals explored how HaystackID® Core Intelligence AI™ and its generative AI (GenAI) features can reshape electronically stored information (ESI) discovery. Moderated by John Brewer, HaystackID’s Chief Artificial Intelligence Officer and Chief Data Scientist, the webcast focused on improving accuracy and efficiency across tasks like first-level review. During the webcast, the speakers highlighted how GenAI streamlines quality control (QC), allowing teams to identify inconsistencies and save time quickly. Despite GenAI’s power, human oversight remains essential to ensure thorough review. Read the full transcript to uncover practical tips for refining your workflows and achieving better results in ESI discovery.
Expert Panelists
+ Esther Birnbaum
Associate General Counsel, Interactive Brokers LLC
+ Jim Sullivan
Founder, eDiscovery AI
+ John Brewer
Chief Artificial Intelligence Officer and Chief Data Scientist, HaystackID
+ Young Yu
Vice President of Advanced Analytics and Strategic Solutions, HaystackID
[Webcast Transcript] 6 AI Workflows to Enhance ESI Discovery
By HaystackID Staff
During a recent HaystackID webcast, legal leaders gathered to explore how HaystackID® Core Intelligence AI™’s generative AI (GenAI) capabilities streamline the review process and enhance accuracy across six critical workflows. Moderated by John Brewer, Chief Artificial Intelligence Officer and Chief Data Scientist at HaystackID, the expert panel highlighted how Core Intelligence AI optimizes workflows for:
- First-Level Review
- Quality Control (QC) of First-Level Reviewers
- Multi-Language Review
- Privilege Review
- PII/PHI Identification and Extraction
- Reviewing Received Productions
Attendees gained practical insights into how GenAI delivers more consistent and accurate results than traditional technologies. As HaystackID’s Young Yu, one of the featured speakers, emphasized, “GenAI does a better job than any of the previous tech out there. It’s a better means of classifying documents. You’ll get more accurate results; you’ll get more consistent results.”
One of the key takeaways from the workshop was how GenAI can revolutionize the quality control (QC) process. Instead of relying solely on manual review for QC—a time-consuming and often inconsistent method—legal teams can leverage GenAI to evaluate document coding across large review teams. This GenAI-enabled QC ensures that inconsistencies are detected quickly without compromising quality.
“You can take a random sample of 500 docs from each reviewer, run it across them, and see which reviewer is coding documents incorrectly in a matter of 20 to 60 minutes [with GenAI],” panelist Jim Sullivan shared during the webcast, underlining the efficiency GenAI brings to the table.
Despite GenAI’s advanced capabilities, the speakers reinforced the importance of human oversight in the eDiscovery process. While GenAI can handle large-scale document processing, human reviewers are crucial in QC and final decision-making. As panelist Esther Birnbaum explained, “There always needs to be extensive QC. That doesn’t change from human to GenAI, and there will always be a human element.”
Read the full transcript to discover how to leverage these insights and actionable strategies to optimize your eDiscovery workflows.
Transcript
Moderator
Hello everyone, and welcome to today’s webinar. We have a great session lined up for you today. Before we get started, there are just a few general housekeeping points to cover. First and foremost, please use the online question tool to post any questions you have, and we will share them with our speakers. Second, if you experience any technical difficulties today, please use the same question tool, and a member of our admin team will be on hand to support you. And finally, just to note, this session is being recorded, and we’ll be sharing a copy of the recording with you via email in the coming days. So, without further ado, I’d like to hand it over to our speakers to get us started.
John Brewer
Thank you very much, Mouna. I am John Brewer. I’m the Chief Artificial Intelligence Officer and Chief Data Scientist at HaystackID. Today, I have a very talented group of folks with me. We’re going to be discussing generative AI (GenAI) workflows, enhancing eDiscovery processes over the next couple of years, and how that’s going to play out and how we see those working. So, if I can get the first set of slides here, that would be great. I’m John Brewer. I’m the CAIO at HaystackID. I’ve been operating in the data space since about the late ’90s, and I have been operating with HaystackID in one capacity or another since about 2015. So it’s been almost 10 years now. And I’ve had the opportunity to actually lead a lot of our processes and our development in the GenAI space. I’m looking very much forward to talking about some of that today. If I can get the next slide here. I would like to introduce Esther Birnbaum, Associate General Counsel from Interactive Brokers. Esther, do you want to give us just a few sentences of biography here?
Esther Birnbaum
Sure. Like John said, I’m an AGC at Interactive Brokers, where I head up eDiscovery and many other areas related to law and technology. I’m really excited to be on this panel because I’ve worked with all the other people on it, so I’m excited for our conversation.
John Brewer
Excellent. Thank you very much, Esther. Please move to the next slide so that I can introduce Mr. Jim Sullivan, founder of eDiscovery AI. Jim, can you take a minute to introduce yourself?
Jim Sullivan
I’m the founder and chief nerd at eDiscovery AI, and I’m here to build AI tools. I have a long history in discovery using predictive coding and analytics, and I’ve spent a decade using predictive coding. I see a lot of possibilities with some of these new AI tools, which I think will really change how we do everything.
John Brewer
Excellent. Thank you very much, Jim. If we can get the next slide here, I will introduce Mr. Young Yu. Young, tell us about yourself. Unfortunately, Seth Schechtman was not able to join us. He had a last-minute complication that prevented him from jumping on the call, but we thank him very much for the time he committed to the prep for this call. If we can get the next slide, I will introduce Mr. Young Yu. Young, tell us about yourself.
Young Yu
Thanks, John. I hate to admit this, but I’ve been doing this for 25 years, and it sort of covers the spectrum here: law firm side, software provider, and vendor side. Probably shouldn’t admit that I like to do math in the background here, but that’s about it. John also has the title of chief nerd here at HaystackID. I’m in second place here.
John Brewer
That’s not official. Okay. So this team has both worked independently and we’ve worked together on various Generative AI projects in qualifying some of these technologies. So one of the primary use cases that have emerged for Generative AI, and I think that this was something that people saw pretty much from the drop when Generative AI kind of burst onto the scene towards the end of 2022 or early 2023, is first-level review. So if I can get the first slide here. First-level review is basically the initial review for relevance and issue-tagging in documents. Now, historically done by human beings, teams of people, who have been going through many, many documents with a rubric to determine whether or not a document was responsive or whether or not particular issues were present in a document so that it could be moved on to the legal team to actually analyze those documents either for production or for internal strategy conversations. We have seen a lot of work go into first-level review and how GenAI performs on it. Now, I definitely have my own thoughts on this, but I think I’m going to start with Young on this one since I know you’ve been doing some studies on how first-level review works. What has your experience with first-level reviews so far, as opposed to other techniques you’ve used?
Young Yu
The biggest difference here is benchmarking, which refers to industry standards for linear review, TAR 1, TAR 2, and now GenAI. The unspoken elephant in the room is that first-level review is probably going to be a very large portion of that budget. No matter how you tackle the documents, you have to review them. I do think that GenAI provides us with the opportunity to cut costs and review fewer documents. The thing that I think differentiates the most here from, let’s say, linear or TAR 1 or TAR 2 is that you do get the logic or the rationale through GenAI. So, in traditional human review, you’re getting a call for responsiveness, you’re getting a call for issue tags, but there is no reasoning behind it. You get footprints in the sand, so to speak. The differentiator here for GenAI is that you get the rationale. It’s important to check the rationale, but you do get the rationale, and you can either take it at face value or dive in, but that is an important piece to this. Speed is the next part. You can scale up or down with reviewers to cover your time spread, but with GenAI, that doesn’t really factor into that equation. You can point it at documents; as long as your prompt is good, you can just let it loose. You don’t have to worry about bringing on a hundred reviewers, multiple training sessions, and then inconsistencies or human understanding of coding protocols; they vary from person to person. And I think the biggest takeaway here is that you’ll have far more consistency when you leverage something like GenAI.
John Brewer
That’s fair. No, I think that’s a good point that you bring up around benchmarking, specifically as you said, but also consistency, which I know has been a contentious point when I’ve heard in the past. I think that there is an assumption by people working in human review who are used to doing the regular review that we do have relatively consistent reviews from humans, which I understand is not necessarily the case, and I think that we have some data to support that. But also, just from an experience perspective, Esther, as somebody who has actually been doing reviews both pre-GenAI and now, actually has a fair amount of experience doing reviews with GenAI, can you give us some feeling about what the difference in experience from the legal end is in first-level review?
Esther Birnbaum
Yeah. So, I think you need to take a step back even before we talk benchmarking. I’ve always believed that very often, human document review for the last decade or two has been set up; I wouldn’t say for failure, but definitely not for success. You hire contract reviewers, and very often, the law firm or even the end client who needs to do the review; they’re not seeing the resumes of these people. They don’t know any of these contract reviewers. We’re trusting our LSPs to give us first-level reviewers and we don’t really know what we’re going to get. And then we sit them down. It used to be in a room, now it’s usually on a call like this where an associate from a law firm spends an hour basically telling them all the information they need about a company and a matter. I work in financial services, and our matters are not easy to understand. And we give them a protocol; we expect them to read it, understand it, and then go into documents and correctly issue tag for 12 different issues I just think it’s an unrealistic expectation, and so we are used to results that aren’t perfect or near perfect. There’s a pretty low bar for what we expect from human review. I think Young probably knows what the statistic is. It’s 70-something, maybe? Jim, maybe? One of you knows for sure. We’ve talked about it.
Young Yu
Anecdotally, I think the odds of getting humans to agree objectively in a space, it’s like 65% of the time. They will agree 65% of the time. Just think about-
Esther Birnbaum
It’s a pretty low bar.
Young Yu
Right, but it compounds when you add more people. You think about meeting up with your friends, and you have five friends, and you want to decide where you want to go to dinner; how many agreements will you get there? Now, if you give them choices, I think it becomes tighter. The fewer choices, it does become tighter. If you gave them two choices, you might get more agreement, but it’s still very difficult to get people to agree a hundred percent of the time.
Esther Birnbaum
Yeah, and I’ll be honest, the document reviews I’ve been involved in at my company, I’m not the subject matter expert, and I even have a hard time understanding, and I spend a lot of time trying to understand exactly what we’re looking for. And I think we’re asking a lot of contract reviewers who don’t have necessarily have backgrounds in the area. When it comes to GenAI, John will make the point over and over that it works on a certain set of documents. And very often that set of documents is the same set that humans are reviewing. You’re not getting much from a human review of a CSV file with millions of rows, et cetera, or other things I’m sure we’re going to remove from a review population. But you do have to go into a GenAI review with a clean document population, which I think is parallel to what you do for a human review because I will never send documents to a human review team that’s not a clean set. And then once you run a GenAI review, I mean, I was blown away by the results. Everybody on this call saw my reaction to our results. It’s much more accurate than humans. And, like Young said, you get an explanation. And even when our precision rates were lower than expected, very often we were able to identify why, or a subset of documents that, oh, maybe these shouldn’t have been run through GenAI, or we can automatically mark all of these relevant because the GenAI is coding it consistently unlike humans who often don’t. When I was a document reviewer back in the day, I would review documents and watch TV. I’m just being honest, don’t tell anybody. I mean, it’s just a whole different ballgame. You get a set of documents, they’re coded, you have a rationale, you have a document summary, and you are able to categorize documents from it. So, for me, it’s not even a question of what I want to do in the future.
John Brewer
No, I think that that makes sense, and absolutely, I suspect that there’s just not enough conversation about the human factors issues with humans. I think that there’s definitely no question of humans not understanding what they’re doing or not being right about it. But when you’re sitting down and you’re reviewing documents for eight hours a day, day after day, night after night, there are limits to attention and other factors with humans.
Esther Birnbaum
It’s not as easy as it sounds.
John Brewer
No, absolutely not. So, Jim, I know you were in Generation Zero of the GenAI revolution in legal tech. You were one of the people who saw the tech coming down and said, “Ah, I see how this can be used.” Right from the jump, you were out there, and you decided that a relevance review was the first objective of this technology. What was the thinking behind saying this? What should it be used for, among all the other things that we’re about to discuss?
Jim Sullivan
There’s a couple of things. Obviously, how much money, effort, and time go into that phase, but the real reason is that it’s so good. It’s just so much better. When it really comes down to it, I struggle to find anything that humans can do in first-level review classification that the AI can’t do incredibly well, that they can’t do better. When we look back at the days of predictive coding of TAR, there are a lot of holes and weak spots. There’s foreign language documents. There’s identifying key documents. There’s identifying narrow issues, hot docs, and different data types. All have struggled where there is just nothing that the humans can win on with a first-level review when you’re comparing it to GenAI, whether it’s foreign language docs, images, different file types. I would put an AI review against a human review any day of the week for any single potential issue that you’re looking for on first-level review.
And that’s what I think the difference is between previous technologies, different things where this is just better and the justification for using human starts to drop, where we see humans consistently getting 70% to 80% of the relevant documents in datasets and GenAI is consistently getting 90% or higher. And we’re not seeing the weak points of being unable to get us our key issues, not being able to find hot docs, not being able to find really rare low-prevalence problems, the foreign language, dealing with images, dealing with audio. All those things that used to be real reasons why predictive coding couldn’t work, or technology wouldn’t be as good as people are just getting to a point where there’s nothing left. And what is the justification for using AI instead of humans? The speed’s always going to be there, the cost is always going to be there, but when it really comes down to it, it’s just the fact that the humans are not as good and there’s nothing that the humans are going to provide to you that the AI’s not. One of the things that we struggle with is how can you measure the accuracy of a system when you’re measuring it with humans as your gold standard? Because what we constantly find is we have a subject matter expert that reviews documents, and then we have AI that reviews the documents, and then when we look at them and, we find documents that are the false positive and false negatives, more often than not, it’s after reading the justification you realize the AI was right. And that’s I think just getting to a level that humans can’t compete with now, they won’t be able to compete within a year, and it’s not going to get closer as we move forward.
Esther Birnbaum
Also, I don’t think it’s comparing equal things. The capability that Jim mentioned that humans can’t do, very often, is translation. I mean, not even just the translation, the review of foreign language documents with GenAI, it does what humans can’t do. It codes them and gives explanations even if they’re in a different language. You’re getting that in English. It’s just mind-blowing. And then the images-
John Brewer
We’re going to get to that.
Esther Birnbaum
Oh, sorry.
John Brewer
No, it’s okay. It’s absolutely a good use case, but I like the foreshadowing.
Young Yu
So there is also, Jim, I’m not trying to counter what you said here, but the prompt building, figuring out the criteria for the prompts, is driven by humans, by subject matter experts, or what have you, and then there are documents that fall outside of that strike zone. I understand that the diminished need may be moving forward for first-level review, but I think that’s always going to be a necessary factor. And again, I know, John, the topic here is first-level review, but let’s be open about this. I don’t think the general public will jump in feet first and say, “Hey, we’re going to do first-level review a hundred percent through GenAI.” I know that’s what we’re speaking to now. I think early adopters will be, “Let’s kickstart active learning models, or let’s use it to backstop.” And I think that bleeds into John’s next slide here. But I do think that the human factor, at least very early on, will be a driver of the methodology and the tech because, as you said, there’s a gold standard, and it’s human review.
John Brewer
So, actually, Young, I think that’s a great transition point. If we want to go to our next slide here, we’re going to be talking specifically about the QC of first-level review. And this alludes to Jim’s point about when we compare results from the AI to results from human review, when we’re doing our orientation, our test scores. We frequently find that the AI may have actually made a better ruling on a given document there. Sorry to cut you off there, Young, Esther. Do you have any input that you want to put in there?
Esther Birnbaum
Yeah, I mean, I agree with Young for QC of first-level review is incredibly important. We’re not taking humans out of this. When I say first-level review, I mean a linear first-level document-by-document review. Now, I think there always needs to be extensive QC. That doesn’t change from human to GenAI, and there will always be a human element. But I’m saying, if we have a million documents, I don’t want a human to go through a million documents to make calls. I want to run it through GenAI and have humans QC that work.
Young Yu
No, and I agree with both points. I think to classify documents as relevant or related to an issue, I think GenAI does a better job than any of the previous tech out there. And not to misclassify human review as tech, but that methodology, I do agree, GenAI, to the extent that you have good prompts, to the extent that you’ve done the homework, I do think it’s a better means of classifying documents. You’ll get more accurate results; you’ll get more consistent results.
Jim Sullivan
Young, is there something that you can think of? What justification would you say would not be for using this as a first-level review tool? What piece is not there that you’d feel would need to be in order to say flat out that this is an absolute replacement in all situations?
Young Yu
You’re putting me on the spot here, Jim. I would say-
John Brewer
I would jump in and say that we definitely have blind spots in different media types right now. We do have tools that can do images. We have tools that can do audio. I have tools that can do video, which, as Esther alluded to earlier, is kind of a side case from a sheer percentage perspective, and actually, also riffing off Esther’s earlier comment, very large document sets. Now those go into structure review anyway. We can argue there is no first-level review for databases and things like that, but that definitely ends up outside. Although I’m curious, Young, can you come up with something that doesn’t use all of the caveats that I just took away from you to answer this question?
Young Yu
I wouldn’t say that there’s a single review that GenAI wouldn’t be helpful; I think it’s more portions of each review. So John alluded to overly-structured data and under-structured data. But aside from that, I think you’re right where GenAI would be helpful in almost any review, but there are going to be portions of that dataset that GenAI is not going to be able to analyze. I don’t disagree that this does give you a leg up in even the most difficult situations. And if cost wasn’t a barrier to entry, even very, very, let’s say, low-richness matters, you just throw it at everything. But that all being aside, let’s just put everybody on equal footing; cost isn’t a factor, let’s say time isn’t a factor, and I’d probably leverage GenAI on everything. I’m not saying that it’d be a hundred percent right. We know that humans are not going to be a hundred percent right, but I think there will be a proportion of documents in every dataset that will require human review. And for the next iteration of GenAI, I think it’s going to probably get to the point where we’re going to run out of things that GenAI can’t classify. We’ll get there when we get there, but in the meantime, as a stopgap, we’re probably layering human review over here. And I’m not saying that’s perfect methodology. I don’t think any of the methodologies are perfect. It’s always been a balancing act whether you’re balancing precision and recall or time and effort. But if all those things are on equal footing here, I wouldn’t find a situation where I wouldn’t be able to leverage GenAI. So, Jim, I’m pointing out to you that I think I’m going on mute for a little bit here. Thanks.
Jim Sullivan
All here together, right?
John Brewer
Yeah. So one thing that I will say that we have seen, and this actually has to do with documentation of data, which is a whole different soapbox that I will crawl on some other day in another master class, is that GenAI is limited to only looking at the document that it’s currently looking at. It doesn’t remember anything that it saw before, and it’s not going to compare it to anything that it’s about to see, which is a great property from an abstract perspective. It doesn’t let itself get impacted by other documents. But that can cause it to have some limitations, not necessarily in the review itself, but in going back to its review manager and saying, “Hey, I think that these were related. They might not have been.”
And the place where we usually see that is something like SMS messages or chat messages or Slack messages where they were broken up in a way that didn’t make sense for the workflow. So, by default, when you see an SMS or something in a review, it’s one day of the conversation between two people. But if that conversation happened very slowly over a couple of days, we would have a whole bunch of documents with one message each. One of the issues with GenAI is that it can’t make those connections across separate documents. We can argue that that’s a shortcoming of how we document and thread the data, whereas a human reviewer would say, “This is clearly stupid. Please find me a new way to review this data.” GenAI just doesn’t talk back the way a real, authentic human does. It doesn’t give you sass.
Jim Sullivan
That feels like a pro than a con.
John Brewer
I’m trying not to put moral judgment on that. But yeah, no, I mean, one I really stress, based on how the technology has developed, that’s the only case. And it is kind of a weird corner case, and arguably, it’s not even in the review phase. It’s almost pre-review, but-
Esther Birnbaum
Well, it’s the same problem with human review. I mean, now we’re better with threading chats, et cetera, but I remember doing a 70,000-document review of Bloomberg Chat, and the only way they could export it, this was a while ago, was one chat per document. So there was no context there either. So, I think a lot of our issues with GenAI can be related back to similar issues with human review. But the other use case or question is, what about document families? Do we want GenAI to look at full families, or are we going to get skewed results if it looks at every document individually because then you don’t have the context?
John Brewer
Right. And is it even the role of first-level review to provide that context?
Esther Birnbaum
That’s an argument we have about it all reviews, right?
John Brewer
It’s true.
Young Yu
There are times when inference can be beneficial and also detrimental, right?
John Brewer
It’s true.
Young Yu
I mean, there’s an inclination that inference is beneficial when reviewing for privilege, like cover email being privileged. You’re going to withhold that email. You’re going to withhold any of the attachments. Now, do you want a call on that parent email, or do you want a call on that family? I think it’s really a preference point. Because let’s say there’s correction involved here, and let’s say you say, okay, the cover email is not privileged, or let’s say we are going to produce in redacted form, what are you doing with the attachments at that point? So that inference can be detrimental in correction because-
Esther Birnbaum
Well, we’re not talking about privilege review.
Young Yu
Right.
Esther Birnbaum
Relevance is very different.
Young Yu
Well, understanding-
Jim Sullivan
Hey, that’s number four here. Young, you can’t jump to slide four. We’re on slide two.
John Brewer
I was about to say-
Young Yu
This is why you don’t show me-
Jim Sullivan
We’re not doing slide two very well.
Young Yu
This is why you don’t show me the deck before we go: I’m jumping all over the place.
John Brewer
We began here anyway.
Esther Birnbaum
I should have looked at the deck before we started.
Young Yu
Well, okay, so let’s stay on topic. I’m sorry that I jumped ahead here. All right, QC of first-level review. I think with every single review, there is a proportion of not responsive documents, whether it’s 10%, 5%, 1%, whatever it is that’s been reviewed in one way, shape, or form, whether it’s been classified as not responsive by let’s say any of the TAR methodologies or by linear review, typically, you still sample that during a second-level pass. And you say, okay, let’s say 5% of the NR documents are going to be QC’d to see if anything was missed. I mean, I think that’s a great place that you can leverage GenAI if you’re looking to get your toes in the water. It’s an easy place to start. It’s not super cost-prohibitive unless you have a giant population. But you can point it at that set, provide your criteria for responsiveness, and say, “Hey, double-check these documents for me. The coding is the coding.” And you can see what was missed, or maybe nothing was missed, but it’s an easy place to slot it in. You can also do that on your responsive docs. So, documents that are in that, if you’re using active learning, let’s say, anything in that lower rank series if you really want to go through and fine-tune. But there are easy ways to slot in GenAI if you’re just looking to get started. And I think that’s where the industry is going to go, where can we find a place to slot in GenAI without either being the first and setting precedents or having to disclose that this is the way we went and exposed the methodology.
John Brewer
I think that’s actually a great segue into our next piece here just because I think that the goal here was to level set with first-level review because that’s the technology that is out there; it is in production at this point. This is what we’re using. Now, we’re talking a little bit more about where the technology is going and what the new workflows are going to be looking like. Let’s get the next slide here.
Jim Sullivan
John, I just want to say that I feel like one of the biggest use cases here is QCing your reviewers on those large matters. I know that no one’s QCing the reviewers as much as they claim they are or as they should, and how often they should is probably something we could debate. But as a matter goes for nine months, two years, five years, I mean, some of these go for a long time with a large team, and most of the time, you’re QCing your reviewers on an individual level at the beginning of your review. They’re going for a week or a month, and you’re taking a random sample of every reviewer’s docs. You’re reviewing to ensure your reviewers understand the issues and that no one’s completely off track.
But as the review goes on, you get concept drift. Every reviewer goes in different directions, and we’re not following up with them or double-checking them. In fairness, you could probably put your feet up, close your eyes, and click on some of these large reviews and not ever get caught if you’re doing it after that first week of checks. Now, with GenAI, I can take a random sample of 500 docs from each reviewer, run it across them, and see which reviewer is coding documents incorrectly the most often in a matter of probably 20 to 60 minutes of effort. You know how long it takes to QC a review team when you have 20 reviewers and you’re reviewing a random sample of each of them. And that’s why it doesn’t get done. This is just such an easy shortcut to providing a higher-quality output for your review and telling your clients that you’re making sure that all your reviewers are on the same page on a regular basis, whether it’s weekly or monthly. Just doing the things that make your review more consistent, which we should be doing on every review that goes out, makes that step easier.
John Brewer
I think that that’s a good point.
Esther Birnbaum
Jimmy, the point you made is really one of the biggest pitfalls of human review is that you have a team of reviewers, and by the end of day one, they’ve reviewed thousands of documents, and you might have one or two associates on a matter who are supposed to QC to provide further direction, and they can’t keep up. It is impossible. And if you’re not getting the feedback to the review team overnight, then they start another day coding incorrectly if you have to shift gears or something. So it’s an excellent point.
John Brewer
Fair. No, absolutely.
Jim Sullivan
Thank you, Esther.
Esther Birnbaum
You’re welcome.
John Brewer
Yeah, no, actually we’re getting back to one of the points Esther raised earlier, which is multi-language review and the fact … Now, this is near and dear to my heart because the way that transformer technology that all of this is predicated on was originally developed was largely around translating from one language to another. And so it’s almost uniquely well-suited for it, although obviously, the massive majority of the corpus that all this was trained on was English. And so we do get really good performance out of English models. I don’t speak enough other languages to really be able to compare myself to say how good other language models are into this anecdotally. But Esther, do you have some thoughts that you wanted to share with us in terms of how you’ve seen foreign language documents get handled by these models?
Esther Birnbaum
Yeah, it’s pretty incredible. I mean, when we’re doing just document review in the way that we’ve done it with GenAI, you’re not getting a translation of the documents, although you can with GenAI, it’s basically just coding it as if it’s English. That’s how it seems. It’s giving you the same document summary and the same issue analysis. One of the document reviews we did involved Hebrew-language documents that were coded. I speak enough Hebrew to check if the coding was accurate, and I was just blown away by the way it did it. I mean, it’s like my nightmare: You finish a review, and then you have these different buckets of categories that we still have to deal with, like foreign language, technical issue, whatever it is. I think that we’ve gotten a lot better at dealing with that on the front end, but just being able to have documents coded like this is just, I mean, finding foreign language reviewers is not easy. So, instead of having to find a team of foreign language reviewers, you find one who can then QC the results from GenAI. It’s a game-changer.
John Brewer
So something just occurred to me and, Young, actually, I think you might be able to answer this for me. How, historically, have we handled multi-language review in TAR?
Young Yu
So, this is a loaded question, but thank you.
Jim Sullivan
Thank you for asking Young. That’s my nightmare right there.
Young Yu
Yeah, no-
Jim Sullivan
Young, take it away.
Young Yu
It’s driven by proportionality. So if you have a document population that’s split 50% English and let’s say 50% Chinese, if you have enough documents that are Chinese language coded, the model should be able to make that distinction. But then again, you still need native reviewers.
Now, as the number of languages increases and scales down with proportionality, let’s say you have six languages and one language is just very discrete, let’s say less than 5%; those documents will probably not be classified correctly unless you code enough of them. So that’s the difficulty here. When we assess a population for its aptitude in an active learning model or TAR 1.0 methodology, whatever we’re going with, you look at the language breakdown, and you see if it makes sense to leverage whether it’s any of the available tech. They’re all supposed to be language agnostic and accepted tech, but at the end of the day, context is everything. And if you’re not providing enough context to form a decision on any one of the languages, you’re not going to be able to classify. We’ve done multiple active learning reviews, and I think the largest one we did in terms of the number of languages was six. However, the proportionality was such that no language overpowered the other, and that worked out fine. We tend to siphon or push document populations off when the proportionality says you’re going to have to code way too many documents for this language to even make sense.
Then, you have to think back to the entire model. If you’re coding a ton of documents in this one language just to make firm decisions in that language, how is it impacting the rest of the documents in all the languages? So it’s better to cordon that off. And whether you put it in its own model or have that native reviewers go through that set. It’s weighing time, benefit, cost, and speed. So I hate giving an. It depends on the answer, but proportionality will decide whether that population will fit neatly into a TAR 1 or TAR 2 methodology. And Jim, like you said, you’re happy I fielded this question. Looking back on our one days, we would always exclude foreign language docs very early on. Very early in active learning, we would exclude foreign language docs or build a separate model just for each foreign language or the predominant foreign language. Right now the alternative to that is machine translation of your foreign language documents and feeding the translated text in. But again, depending on the translation method, you lose context. I don’t know that there’s a perfect way to do this. I think GenAI does provide us the opportunity to have more insight and better classification. But at the same time, without that end translation, when you’re producing a document or you’re taking a document forward to senior counsel, an SME, or an SME group for litigation or depo prep, that translation is going to matter, right? So again, I think it’s sort of-
John Brewer
Yeah, I think those are very good points.
Young Yu
Right.
John Brewer
So I was going to say, so Jim, would you feel comfortable doing multi-language reviews in our current technology or do you think that’s something that needs more development before it’s ready for prime time?
Jim Sullivan
I think the current GenAI is incredible at foreign language, and I think one of the easiest use cases that we recommend is what happens when you have that small set of very difficult language. You have a few thousand documents that are in Japanese, Chinese, or Korean. This is a really easy solution to run it through, get a summary, see whether it’s related to your issues, and then determine what to do with them next. Going out and finding that reviewer or trying to get a machine translator to see what you’re dealing with is just a little bit of a longer process that’s not nearly as accurate. All the things that Young mentioned about handling TAR and training models on different languages were just a really big burden. Usually, what would happen on a TAR 1 approach when we’re trying to train different languages is that they’d give up and not be able to train one or two or three or all of the languages well., I feel like this is a really easy entry point for some users to get some really good summaries on a small set of foreign language documents and understand what they are dealing with. Again, it’s low risk, like the QC, where this is just giving you information that you wouldn’t have been able to get quickly in the past.
John Brewer
Excellent. No, I think that’s a good point. Let’s get the next slide up here because I think we need to talk about privilege. Now, Esther, I think I poked you earlier in our preparation to help give us some context around the difference between relevance review and privilege review in a practical sense.
Esther Birnbaum
In a practical sense, relevance review is used to determine what documents are substantively relevant in order for us to produce them. Privilege is to ensure that from the relevant documents, we are not producing any information that falls under any type of privilege.
John Brewer
And so I think I know that from a nerdy, computer-science, data-science perspective, I was like, “Well, that means that precision is going to matter a little more than recall in calculating the output of this.” In a real-world sense, what are the stakes? If you can, just put that in context: if we mess up a relevancy review, we don’t produce a document, and our score goes down. And we’ve had conversations about Peck standards and things like that to make sure that we produce the adequate amounts. What are the real-world implications for a case if the privilege review goes poorly?
Esther Birnbaum
It’s really bad, but I mean first of all, you’re releasing information you do not want to, that can be held against you. I mean, you can do claw-backs, et cetera, and when you’re writing your ESI protocol, take that into consideration. However, there are also situations where you waive privilege when you produce privileged documents, which means that the opposing side can ask for a lot more privileged documents.
I would say that producing privileged documents’s at the top of the list of lawyers’ nightmares about document review. I don’t know if we want to tie this into confidentiality in a similar way. Still, there are both producing privileged or producing confidential documents that you shouldn’t have serious implications.
Young Yu
The over-assertion of privilege is just as bad, though, Esther, right?
Esther Birnbaum
Yeah.
Young Yu
So getting challenged on your privilege log and then having to go and re-evaluate documents for over-assertion of privilege. And I always thought that attorneys’ nightmares about document review was doing document review. That’s not true.
John Brewer
So yeah-
Esther Birnbaum
I don’t think there’s a difference between doing a relevance review.
Young Yu
No.
Esther Birnbaum
I’m not going to lie that I had fun QCing our GenAI review results. But yeah, I definitely don’t want to be doing a document-by-document review. But yeah, I mean, in terms of over-asserting privilege, it’s a balance. You don’t want to make people annoyed. You don’t want the judges to be annoyed, you don’t want [inaudible 00:44:48] to be annoyed by your privilege calls being challenged, but at the same time, privilege isn’t objective. In law school, very often, you learn the classic privilege cases is an employee operating with their legal hat or their compliance hat, and that’s something we deal with every day. Five different people will have different opinions, and there’s just not always an objective answer, which is why I think GenAI is going to be incredibly helpful with privilege review, especially with entity identification, rationale, and explanations. But there will always be a human element to all of this because not every call is objective. So we can just have AI answer.
John Brewer
So, Jim, I’ll throw it to you in just a moment, but I have one question for Esther after that. Having established the stakes and why privilege review is an in-kind difference from relevance review, you have been extremely bullish on using AI for first-level review. Do you feel the same way for privilege review?
Esther Birnbaum
I’ll be honest. Are you asking Jim or me?
John Brewer
I’m asking you first and I know what Jim’s answer is going to be. I think I do anyways, we’ll find out. But how do you feel about putting a GenAI on your privilege review?
Esther Birnbaum
I feel very positive about it. I haven’t really done it yet.
John Brewer
Fair enough.
Esther Birnbaum
It’s just been released recently, and Jim can probably talk about that more. But based on what I’ve seen—I mean, again, the way GenAI is able to classify and give rationale—I just think it’s going to be really helpful across the board.
John Brewer
Okay. Absolutely. Okay. And I’m sorry, Jim, I know you’ve been waiting patiently.
Jim Sullivan
It might come as a surprise to say that I don’t see GenAI being a replacement for human analysis on privilege. I see GenAI as being more of an assistant role to help you make sure that you’re not having the nightmares Esther’s talking about where you’re missing something. We’ve all used a lot of different techniques to identify and flag potentially privileged documents from keywords and use analysis to help flag things that are potentially privileged. I think GenAI is the best tool out there to flag things that are potentially privileged. And then using that as just another tool to make sure that you’re not missing something is incredibly valuable. I don’t know if I would go out there and tell people that this is a tool that you should run across all your data and completely rely on the results for privilege because I think that we’ll still need to do more testing and make sure that that’s still the best case. Now, it’s absolutely the best tool out there, but I still think we want to have that human element in play and just having it flag things that a human might’ve missed or creating a priv log entry might be the most valuable piece of using it. But I would pull back a little bit on my…With privilege, I see it as, at the point where we’re at now, which I absolutely think will change as tools get better and better, we’re at a point where you should use GenAI to flag and possibly identify privileged documents that you may have missed. But it should not be the end-all, be-all reliance on it. I would say I have more confidence with the relevancy review.
Young Yu
Jim’s overriding his programming here, but-
John Brewer
No, I think he’s absolutely right.
Young Yu
No, I agree with that. I agree with that. And you think of traditional methods to sort of pre-screen privilege; it’s typically going to be search terms and/or attorneys that generate privilege. Those are the markers that we work off. We run a broad sweep of search terms and then identify communicators that generate privilege. And most of the time, and forgive me for not having raw stats on it, I would say 60% of that population is privileged on a good day. On a bad day, 15% of that population is privileged, actually privileged. I do think GenAI is a better indicator of privilege. And just like Jim’s saying, the tech is going to be better than just applying privilege terms and identifying individuals that generate privilege. Again, it is backstopped by maybe even overly broad search terms and human review, I mean, whether you use additional search terms or not, just to take a look at outliers. But again, it’s an easy way to get your foot in the water here, right?
John Brewer
Yeah, you’re right. I was going to say we’re truing up our hour, and I want to make sure we have a little time for Q&A here. So let’s go to our next slide, which is very near and dear to my heart.
Jim Sullivan
I mean we all agree on privilege, so it’s not like we should … If we’re not going to fight about it, what’s the point?
John Brewer
Yeah, no, we’ve just spent six minutes agreeing with each other. Well done, us.
Young Yu
That wasn’t planned. That wasn’t planned.
John Brewer
No, I was hoping somebody was, but anyway, PII/PHI. Basically, this is going through a bunch of documents, pulling out all the names of individuals in there, and pulling out all the titles. Like I said, it is near and dear to me because I was at the helm of creating Protect Analytics, which is HSID’s solution and that’s built on the previous generation of AI, which, as you know, machine learning techniques and whatnot. And we’ve definitely been working with GenAI to do some PII and PHI extraction here. I was going to say, Jim, do you have any thoughts on that? I’ve heard rumors that there might be something coming from you on that.
Jim Sullivan
Yeah, this is something we’re working on and this is one of those things that just constantly blows my mind. The accuracy and the quality are just game-changers. I mean, we know the amount of effort that goes into these jobs, the human labor required to extract information like this, and the cost that goes into these PII extractions in data breaches. The ability of large language models to identify and extract PII is phenomenal. It is going to absolutely change the industry, just as much as relevancy review is going to change the eDiscovery review industry, but that’s my highest opinion, maybe.
John Brewer
So, I think the distinction that I’ll put in here is that right now we have extracting PII is almost a solved problem even with last-generation technology. The fascinating thing for me is that GenAI is able to connect pieces of PII, such as this phone number belonging to this person and this social security number belonging to this person. I think that’s really going to be the game-changer. We call it entity extraction in most cases and there are tools out there that do okay with it. But yeah, I think that you’re absolutely right in that the GenAI is going to be a game changer in terms of the quality of those entities.
Jim Sullivan
I think when you compare identifying relevance in a complex document and identifying things that pretty much regular expressions can find in most cases, I just don’t think PII identification extraction is a difficult problem for computers. This is one of those tasks that computers are just very well suited for.
John Brewer
Excellent.
Esther Birnbaum
I have a question for you, John, not to turn it around, but-
John Brewer
I’m the moderator here. No.
Esther Birnbaum
With PII identification, we’re currently working in a time where we have seen lots of rules from many different regulators including the SEC, about the timeframe where you would have to report. I know that right now, you guys are able to do a sensitive data assessment pretty quickly, but do you think that GenAI is going to help that process, make it maybe more robust and quicker in terms of really identifying exposed or breached PII for reporting purposes?
John Brewer
So I would say that I expect GenAI not necessarily to make the initial, the ECA, and the early case assessment phase much faster. We’re pretty quick with that. I think it will be much faster at the second phase of that, which is actually going from an assessment of who’s in the population to what information has been exposed and putting together notification lists and other compliance documents. I think that that second step from an early assessment into actual regulatory action is going to get much, much faster, which is good because the deadlines are pretty tight and some of these data breaches are getting pretty big. Okay, let’s take a look at our last slide here, which, I think, brings together everything that we’ve talked about, which is review and receive documents or receive productions. Basically, taking all of these tools that we’ve used and just using them on the stuff coming in as opposed to the stuff that we’re sending out. Does anybody have any thoughts on how this is distinct and what the kind of factors on reviewing received productions are that are different from the outbound?
Esther Birnbaum
When you were saying earlier, find a use case, I think reviewing a received production is basically a soft launch into a GenAI review because what do you need when you get a production? You need to understand what is in it, and then you need to use it for various purposes, trials, or even so you know what your next discovery requests are or to prepare for depositions, etc. The same way that a relevancy review would classify documents, I think you’re going to get a very high recall and precision rate, I would assume, with a received production. Well, it depends if it’s a data dump or not, but I think it’s a really good use case and soft launch into using GenAI.
John Brewer
I think that’s very reasonable in terms of just getting people in the door. By the way, we’re going into our QA just after this. We probably have time for one or two questions. Make sure that if you have any for our speakers, you submit them using the Q&A button on your Zoom client.
So I was going to say, Young, we used TAR and its various other corollary apps for reviews received. What are the differences when we do that?
Young Yu
We have, and we typically take exemplar documents from our client’s population as seed documents and leverage those against the inbound productions. It sort of stacks up, but when you go back and think about it, what you’ve been asked to produce and what you’ve asked opposing to produce, if they don’t line up a hundred percent, you’re really finding your own documents or your own conceptual documents in opposing.
So long as those production criteria in your document requests line up, it works fairly good. To the extent that they’re very different, again, active learning, you just throw reviewers at it, give them a coding protocol, and get to work. The fear is, just as Esther said, a document dump, you get 8 million documents, and there are only like 300,000 actually responsive documents in that set.
Esther Birnbaum
But it’s helpful to know that off the bat, right?
Young Yu
No, it definitely is. Yeah, absolutely. Because then you can just go ask for relief. You can just say, “Hey, what did you guys produce to us, and why did you do this?” And here’s the burden or whatever that argument is. But in terms of leveraging TAR, if you’ve paid to review your own documents, the real question is whether you are paying to review opposing documents. You’re going to pay, it’s just who’s doing that review and how much time do you have? So when that discovery window closes, how much time do you have to prep for depositions? All this becomes a factor. So like you’re saying, Esther, GenAI is an easy first use case for reviewing received productions. Fair warning though, we’ve had clients come to us with ESI protocols that prohibit the use of GenAI on their productions specifically.
Esther Birnbaum
Wow.
Jim Sullivan
We’ve been seeing more and more talks of that. A question for Esther: When you receive a production like this, how many documents matter? You received a big chunk. How many documents are you using? How many documents are you reallylooking for? In a production, you have to produce everything that meets what they’re describing in your obligation. But for a received production, what’s really the goal, and what are you trying to find, and how many documents does that usually end up being?
Esther Birnbaum
I think it depends on the matter. In some situations, it’s not going to matter that much what you’re receiving, but if you’re, let’s say, dealing with a third-party bankruptcy or liquidation matter, and you’re getting documents from the other defendants in a matter or things like that, then you want to see it. When it’s a single claimant arbitration where they’re basically producing back the documents we’ve sent to them, it’s less important. So I really think it depends. When we’re talking about NDL litigation, it’s probably going to be more important. Different things, it depends.
Jim Sullivan
One thing that I always heard with TAR is that people didn’t like using TAR on the incoming production because they were looking for the smoking gun doc. They were looking for the doc where someone agrees to participate in the conspiracy or something. And that’s what I heard from a couple of people, and TAR can’t do that. TAR can’t find the context. It can’t be understood when you’re subtly suggesting something. And that’s where I think GenAI really stands out.It is able to find your hot docs, your documents that are very, very narrowly tailored towards that one thing that you’re trying to find. And that’s, I think, the biggest difference between how TAR works in finding your key docs where TAR is fantastic at identifying everything you need to produce, but it’s not good at identifying the eight docs that matter.
Esther Birnbaum
When you find those eight documents, and there’s still a huge population of documents out there, and you run an active learning workflow based on those documents, your results are just fantastic. I did that recently.
John Brewer
So I think that we’re actually basically at time now, but thank you all very much for your time. Let’s just say if you have one point that you want people to take away from this conversation, what would it be? Starting with you Esther.
Esther Birnbaum
It would be to stop talking about GenAI and find a use case and see how it works because I just think it’ll change everybody’s mind.
John Brewer
Excellent. Experience trumps all. I like that message. Young, what would your one takeaway that you want people to have from this call be?
Young Yu
I mean it’s echoing Esther’s thought, but sort of on a different line. Adoption with TAR 1 was slow. Adoption with active learning might’ve been a little better, but it was still slow. I really hope the adoption with GenAI is a little more fast-tracked here. I think it is going to change the game. I think the more early adoption we have, the better.
John Brewer
Fair enough. Great. And Jim, one tip-
Jim Sullivan
To echo Esther, it’s like, man, how many times do you sit through a CLE course on these things? Don’t listen to people talk about it. Go out there, run it across some documents, and see what the results are. I mean, it’s just really valuable to get the hands-on approach, and you’re not going to ever get that when hearing a bunch of people sitting around talking about it on a webcast like this.
John Brewer
Fantastic.
Jim Sullivan
But definitely keep coming to these events.
John Brewer
Well, you heard it here: get off this webcast and go do a GenAI project. Thank you all very much for your time. Young, Esther, Jim, thank you so much. Mouna, thank you very much for hosting our call, and thank you to Mary Bennett and Rob Robinson at HaystackID for organizing and coordinating this. And, of course, thank you to all of our attendees who have been with us for the last hour. We greatly appreciate your time. And again, on behalf of HaystackID and Lexology, have a wonderful rest of your day.
Expert Panelists’ Bios
+ Esther Birnbaum
Associate General Counsel, Interactive Brokers LLC
Esther Birnbaum is an accomplished attorney with extensive expertise in eDiscovery, AI, Privacy, and Information Governance. She is currently Associate General Counsel at Interactive Brokers, where she leads the eDiscovery team and oversees the firm’s responses to litigation and investigations by federal and state regulators, exchanges, and self-regulatory organizations. With a robust background in complex litigation and regulatory compliance, she brings a wealth of knowledge and practical experience to the table. She uses her unique expertise at the intersection of technology, data, and law to develop best practices and drive innovative workflows across many areas of the business. She enjoys sharing her insights and knowledge with the wider eDiscovery community, and she frequently speaks at conferences, webinars, and podcasts on topics related to law and technology.
+ Jim Sullivan
Founder, eDiscovery AI
Jim Sullivan is an accomplished attorney and a leading expert in legal technology. As the co-founder of eDiscovery AI, he is at the forefront of transforming how the legal industry leverages advanced artificial intelligence in document review. With two decades of experience, Jim has become a recognized authority on integrating AI into legal workflows, playing a key role in modernizing eDiscovery practices. Throughout his career, Jim has consulted on thousands of predictive coding projects, utilizing AI to efficiently identify relevant documents in complex, large-scale legal matters. His expertise has made him a sought-after speaker at legal technology conferences, webinars, and meetings, where he advocates for the adoption of AI to improve productivity, accuracy, and defensibility in legal proceedings. Known for his forward-thinking approach, Jim encourages legal professionals to embrace AI as a means to future-proof their careers. In addition to his practical contributions, Jim has co-authored The Book on Predictive Coding: A Simple Guide to Understanding Predictive Coding in e-Discovery and authored The Book on AI Doc Review: A Simple Guide to Understanding the Use of AI in eDiscovery, both of which serve as essential resources for understanding the impact of AI in legal practices.
+ John Brewer
Chief Artificial Intelligence Officer and Chief Data Scientist, HaystackID
John Brewer has worked with HaystackID since 2015 and now serves as the Chief Artificial Intelligence Officer and the Chief Data Scientist. In his role as CAIO, Brewer guides the company’s integration of artificial intelligence and generative AI within the legal technology sector, capitalizing on his remarkable two decades of AI experience. He has been pivotal in the adoption of large-scale technology-assisted review, developing the suite of AI-based machine learning tools that power HaystackID’s review process, driving loss prevention, and ensuring unparalleled efficiency – most notably, Protect Analytics, the company’s exclusive set of technologies and processes that allow client data set analysis for sensitive information including personally identifiable information and protected health information, as well as data breach code anomalies. Brewer’s approach avoids opportunistic trends, centering instead on thoroughly researched AI solutions that are in line with the client’s real needs and ethical standards. Brewer’s deep understanding of decades of AI capabilities distinguishes him as an exceptional leader and innovator.
+ Young Yu
Vice President of Advanced Analytics and Strategic Solutions, HaystackID
Young Yu joined HaystackID in 2018 and is currently the Vice President of Advanced Analytics and Strategic Solutions. Prior to his current role, Yu was the Director of Advanced Analytics and Strategic Solutions at HaystackID. In this role, Young was the primary strategic and operational adviser to HaystackID clients in matters relating to the planning, execution, and management of eDiscovery activities.
About HaystackID®
HaystackID solves complex data challenges related to legal, compliance, regulatory, and cyber events. Core offerings include Global Advisory, Data Discovery Intelligence, HaystackID Core® Platform, and AI-enhanced Global Managed Review powered by its proprietary platform, ReviewRight®. Repeatedly recognized as one of the world’s most trusted legal industry providers by prestigious publishers such as Chambers, Gartner, IDC, and Legaltech News, HaystackID implements innovative cyber discovery, enterprise solutions, and legal and compliance offerings to leading companies and legal practices around the world. HaystackID offers highly curated and customized offerings while prioritizing security, privacy, and integrity. For more information about how HaystackID can help solve unique legal enterprise needs, please visit HaystackID.com.
Assisted by GAI and LLM technologies.
Source: HaystackID